AugmentedGaussianProcesses.jl, a full Gaussian Process toolkit | Théo Galy-Fajou | JuliaCon 2020
Gaussian Processes (GP) are an essential model of Bayesian non-parametrics. While multiple GP packages already exist in Julia such as Stheno.jl or GaussianProcesses.jl, AugmentedGaussianProcesses.jl has a larger scope of applications and is constantly updated with state-of-the-art methods. One of its specificity is to work with augmented variables to simplify inference. In this talk I will briefly explain this concept and show the potential of the package.
Started as a very specific research project, AugmentedGaussianProcesses.jl (AGP) is now a package with a much broader range of tools.
A large class of different of problems are implemented such as Student-T likelihood, heterogeneous multi-output GP, heteroscedastic regression. Are also implemented various type of inference like variational inference, Gibbs sampling, Hamilton Monte-Carlo or even variational streaming!
In this sense AGP aims at being a competitor with general GP toolbox such as GPFlow or GPytorch with better or same training and prediction performance.
However one of the additional strength of AGP is to convert problems which start as being intractable into easy ones via latent variable augmentations with a method based on my academic work. It is done so far case by case but current work will aim at being able to treat any problem.
In this talk I will showcase all the potential of AGP, and compare its performance with other Julia and Python solutions. Time Stamps:
00:00 Welcome!
00:10 Help us add time stamps or captions to this video! See the description for details.
Want to help add timestamps to our YouTube videos to help with discoverability? Find out more here: https://github.com/JuliaCommunity/YouTubeVideoTimestamps
Interested in improving the auto generated captions? Get involved here: https://github.com/JuliaCommunity/YouTubeVideoSubtitles
Видео AugmentedGaussianProcesses.jl, a full Gaussian Process toolkit | Théo Galy-Fajou | JuliaCon 2020 канала The Julia Programming Language
Started as a very specific research project, AugmentedGaussianProcesses.jl (AGP) is now a package with a much broader range of tools.
A large class of different of problems are implemented such as Student-T likelihood, heterogeneous multi-output GP, heteroscedastic regression. Are also implemented various type of inference like variational inference, Gibbs sampling, Hamilton Monte-Carlo or even variational streaming!
In this sense AGP aims at being a competitor with general GP toolbox such as GPFlow or GPytorch with better or same training and prediction performance.
However one of the additional strength of AGP is to convert problems which start as being intractable into easy ones via latent variable augmentations with a method based on my academic work. It is done so far case by case but current work will aim at being able to treat any problem.
In this talk I will showcase all the potential of AGP, and compare its performance with other Julia and Python solutions. Time Stamps:
00:00 Welcome!
00:10 Help us add time stamps or captions to this video! See the description for details.
Want to help add timestamps to our YouTube videos to help with discoverability? Find out more here: https://github.com/JuliaCommunity/YouTubeVideoTimestamps
Interested in improving the auto generated captions? Get involved here: https://github.com/JuliaCommunity/YouTubeVideoSubtitles
Видео AugmentedGaussianProcesses.jl, a full Gaussian Process toolkit | Théo Galy-Fajou | JuliaCon 2020 канала The Julia Programming Language
Показать
Комментарии отсутствуют
Информация о видео
29 июля 2020 г. 17:45:01
00:07:52
Другие видео канала
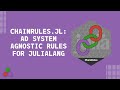


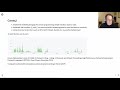
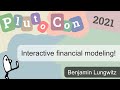
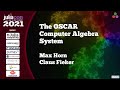
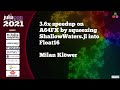



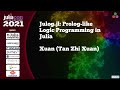
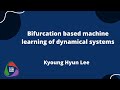
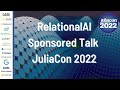
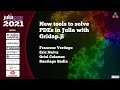
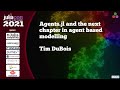
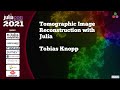

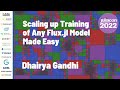

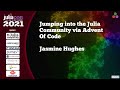