Fighting Cybercrime: A Joint Task Force of Real Time Data and Human Analytics by William Callaghan
Cybercrime is big business. Gartner reports worldwide security spending at $80B, with annual losses totalling more than $1.2T (in 2015). Small to medium sized businesses now account for more than half of the attacks targeting enterprises today. The threat actors behind these attacks are continually shifting their techniques and toolkits to evade the security defenses that businesses commonly use. Thanks to the growing frequency and complexity of attacks, the task of identifying and mitigating security-related events has become increasingly difficult.
At eSentire, we use a combination of data and human analytics to identify, respond to and mitigate cyber threats in real-time. We capture all network traffic on our customers’ networks, hence ingesting a large amount of time-series data. We process the data as it is being streamed into our system to extract relevant threat insights and block attacks in real-time. Furthermore, we enable our cybersecurity analysts to perform in-depth investigations to: i) confirm attacks and ii) identify threats that analytical models miss. Having security experts in the loop provides feedback to our analytics engine, thereby improving the overall threat detection effectiveness.
So how exactly can you build an analytics pipeline to handle a large amount of time-series/event-driven data? How do you build the tools that allow people to query this data with the expectation of mission-critical response times?
In this presentation, William Callaghan will focus on the challenges faced and lessons learned in building a human-in-the loop cyber threat analytics pipeline. They will discuss the topic of analytics in cybersecurity and highlight the use of technologies such as Spark Streaming/SQL, Cassandra, Kafka and Alluxio in creating an analytics architecture with missions-critical response times.
Видео Fighting Cybercrime: A Joint Task Force of Real Time Data and Human Analytics by William Callaghan канала Spark Summit
At eSentire, we use a combination of data and human analytics to identify, respond to and mitigate cyber threats in real-time. We capture all network traffic on our customers’ networks, hence ingesting a large amount of time-series data. We process the data as it is being streamed into our system to extract relevant threat insights and block attacks in real-time. Furthermore, we enable our cybersecurity analysts to perform in-depth investigations to: i) confirm attacks and ii) identify threats that analytical models miss. Having security experts in the loop provides feedback to our analytics engine, thereby improving the overall threat detection effectiveness.
So how exactly can you build an analytics pipeline to handle a large amount of time-series/event-driven data? How do you build the tools that allow people to query this data with the expectation of mission-critical response times?
In this presentation, William Callaghan will focus on the challenges faced and lessons learned in building a human-in-the loop cyber threat analytics pipeline. They will discuss the topic of analytics in cybersecurity and highlight the use of technologies such as Spark Streaming/SQL, Cassandra, Kafka and Alluxio in creating an analytics architecture with missions-critical response times.
Видео Fighting Cybercrime: A Joint Task Force of Real Time Data and Human Analytics by William Callaghan канала Spark Summit
Показать
Комментарии отсутствуют
Информация о видео
Другие видео канала
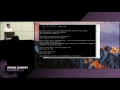
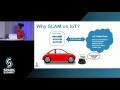


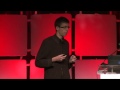
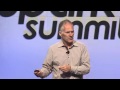

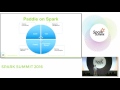
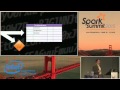
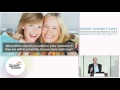
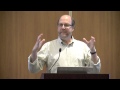
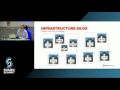
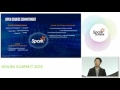
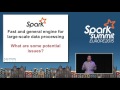
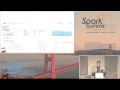
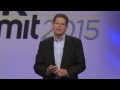

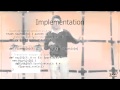
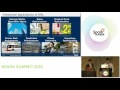
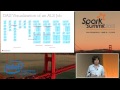
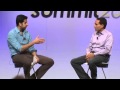