Prof. Sepp Hochreiter: A Pioneer in Deep Learning
Support us! https://www.patreon.com/mlst
MLST Discord: https://discord.gg/aNPkGUQtc5
In this exclusive interview filmed at NeurIPS 2022, Tim Scarfe speaks with Sepp Hochreiter, a pioneer in the fields of machine learning, deep learning, and bioinformatics, and the inventor of the long short-term memory (LSTM) neural network architecture. Sepp shares his insights on topics such as abstraction, uncertainty, and representation in AI, as well as the current state of research in the field.
Sepp Hochreiter is a German computer scientist who has made significant contributions to machine learning and deep learning. Since 2018, he has led the Institute for Machine Learning at the Johannes Kepler University of Linz, after having led the Institute of Bioinformatics from 2006 to 2018. In 2017, he became the head of the Linz Institute of Technology (LIT) AI Lab, and he is also a founding director of the Institute of Advanced Research in Artificial Intelligence (IARAI).
Hochreiter has previously held positions at the Technical University of Berlin, the University of Colorado at Boulder, and the Technical University of Munich. He is also a chair of the Critical Assessment of Massive Data Analysis (CAMDA) conference.
In this interview, Sepp discusses the development of the LSTM neural network architecture, which he invented in 1991. LSTM overcomes the problem of recurrent neural networks (RNNs) forgetting information over time, known as the vanishing or exploding gradient problem. LSTM networks have been used in many applications, such as Google Voice for transcription and search, and in the Google Allo chat app for generating response suggestions.
Sepp also shares his thoughts on the importance of uncertainty in AI models, explaining that users need to know how reliable a model's predictions are in order to trust them. He believes that understanding the uncertainty in models is a key area of research for the AI community.
During the interview, Sepp and Tim discuss the role of abstraction in AI systems, with Sepp emphasizing the need for AI to evolve beyond human-centered definitions of general intelligence. They also explore the idea of representation in AI models, with Sepp suggesting that humans think symbolically and that AI models should incorporate human-intelligible structure to better understand and represent the world.
Sepp goes on to discuss his interest in discrete versus continuous models, stating that humans can only think in discrete terms and that this may be a limitation of current neural networks. He believes that incorporating symbolic quantification and abstraction earlier in the learning process may lead to improved performance in AI models.
Finally, Sepp shares his thoughts on the need for strong priors in AI systems, arguing that humans rely on societal and educational priors to learn and that AI models should similarly incorporate prior knowledge in order to learn efficiently.
This engaging conversation provides a fascinating glimpse into the mind of a pioneer in machine learning and deep learning. Don't miss this opportunity to learn from Sepp Hochreiter's expertise and insights into the future of AI research.
https://www.iarai.ac.at/people/sepphochreiter/
https://scholar.google.at/citations?user=tvUH3WMAAAAJ&hl=en
Interviewer: Dr. Tim Scarfe, CTO XRAI Glass - https://xrai.glass/
00:00 - Start
00:56 - Predictions for AGI
01:41 - Definition of AI
05:32 - Structure at different scales
07:05 - Priors
07:49 - Discrete vs continous
09:19 - Research topics
Видео Prof. Sepp Hochreiter: A Pioneer in Deep Learning канала Machine Learning Street Talk
MLST Discord: https://discord.gg/aNPkGUQtc5
In this exclusive interview filmed at NeurIPS 2022, Tim Scarfe speaks with Sepp Hochreiter, a pioneer in the fields of machine learning, deep learning, and bioinformatics, and the inventor of the long short-term memory (LSTM) neural network architecture. Sepp shares his insights on topics such as abstraction, uncertainty, and representation in AI, as well as the current state of research in the field.
Sepp Hochreiter is a German computer scientist who has made significant contributions to machine learning and deep learning. Since 2018, he has led the Institute for Machine Learning at the Johannes Kepler University of Linz, after having led the Institute of Bioinformatics from 2006 to 2018. In 2017, he became the head of the Linz Institute of Technology (LIT) AI Lab, and he is also a founding director of the Institute of Advanced Research in Artificial Intelligence (IARAI).
Hochreiter has previously held positions at the Technical University of Berlin, the University of Colorado at Boulder, and the Technical University of Munich. He is also a chair of the Critical Assessment of Massive Data Analysis (CAMDA) conference.
In this interview, Sepp discusses the development of the LSTM neural network architecture, which he invented in 1991. LSTM overcomes the problem of recurrent neural networks (RNNs) forgetting information over time, known as the vanishing or exploding gradient problem. LSTM networks have been used in many applications, such as Google Voice for transcription and search, and in the Google Allo chat app for generating response suggestions.
Sepp also shares his thoughts on the importance of uncertainty in AI models, explaining that users need to know how reliable a model's predictions are in order to trust them. He believes that understanding the uncertainty in models is a key area of research for the AI community.
During the interview, Sepp and Tim discuss the role of abstraction in AI systems, with Sepp emphasizing the need for AI to evolve beyond human-centered definitions of general intelligence. They also explore the idea of representation in AI models, with Sepp suggesting that humans think symbolically and that AI models should incorporate human-intelligible structure to better understand and represent the world.
Sepp goes on to discuss his interest in discrete versus continuous models, stating that humans can only think in discrete terms and that this may be a limitation of current neural networks. He believes that incorporating symbolic quantification and abstraction earlier in the learning process may lead to improved performance in AI models.
Finally, Sepp shares his thoughts on the need for strong priors in AI systems, arguing that humans rely on societal and educational priors to learn and that AI models should similarly incorporate prior knowledge in order to learn efficiently.
This engaging conversation provides a fascinating glimpse into the mind of a pioneer in machine learning and deep learning. Don't miss this opportunity to learn from Sepp Hochreiter's expertise and insights into the future of AI research.
https://www.iarai.ac.at/people/sepphochreiter/
https://scholar.google.at/citations?user=tvUH3WMAAAAJ&hl=en
Interviewer: Dr. Tim Scarfe, CTO XRAI Glass - https://xrai.glass/
00:00 - Start
00:56 - Predictions for AGI
01:41 - Definition of AI
05:32 - Structure at different scales
07:05 - Priors
07:49 - Discrete vs continous
09:19 - Research topics
Видео Prof. Sepp Hochreiter: A Pioneer in Deep Learning канала Machine Learning Street Talk
Показать
Комментарии отсутствуют
Информация о видео
Другие видео канала
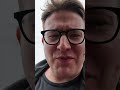
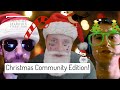
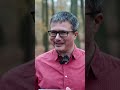
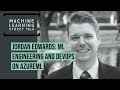

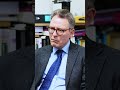
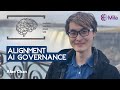
![#62 Dr. GUY EMERSON [Unplugged]](https://i.ytimg.com/vi/dhM0_EKLg9k/default.jpg)
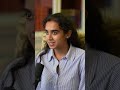
![#83 Dr. ANDREW LAMPINEN (Deepmind) - Natural Language, Symbols and Grounding [NEURIPS2022 UNPLUGGED]](https://i.ytimg.com/vi/46A-BcBbMnA/default.jpg)
![#52 - Dr. HADI SALMAN - Adversarial Examples Beyond Security [MIT]](https://i.ytimg.com/vi/_eHRICHlg1k/default.jpg)
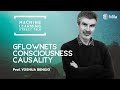
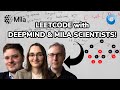
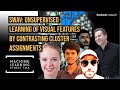
![#71 - ZAK JOST (Graph Neural Networks + Geometric DL) [UNPLUGGED]](https://i.ytimg.com/vi/jAGIuobLp60/default.jpg)
![#85 Dr. Petar Veličković (Deepmind) - Categories, Graphs, Reasoning [NEURIPS22 UNPLUGGED]](https://i.ytimg.com/vi/1lkdWduuN14/default.jpg)
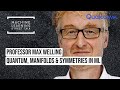
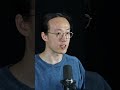
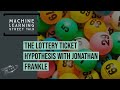
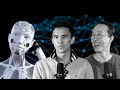