Post-training Quantization in TensorFlow Lite (TFLite)
Post-training quantization is a conversion technique that can reduce model size while also improving CPU and hardware accelerator latency in TensorFlow Lite (TFLite) models with little degradation in accuracy.
Link to the previous video : https://www.youtube.com/watch?v=bKLL0tAj3GE
Link to the notebook : https://github.com/bhattbhavesh91/tflite-tutorials/blob/master/tflite-part-2.ipynb
You can quantize an already-trained float TensorFlow model when you convert it to TensorFlow Lite format using the TensorFlow Lite Converter.
If you do have any questions with what we covered in this video then feel free to ask in the comment section below & I'll do my best to answer those.
If you enjoy these tutorials & would like to support them then the easiest way is to simply like the video & give it a thumbs up & also it's a huge help to share these videos with anyone who you think would find them useful.
Please consider clicking the SUBSCRIBE button to be notified for future videos & thank you all for watching.
You can find me on:
Blog - http://bhattbhavesh91.github.io
Twitter - https://twitter.com/_bhaveshbhatt
GitHub - https://github.com/bhattbhavesh91
Medium - https://medium.com/@bhattbhavesh91
#tflite #Quantiziation
Видео Post-training Quantization in TensorFlow Lite (TFLite) канала Bhavesh Bhatt
Link to the previous video : https://www.youtube.com/watch?v=bKLL0tAj3GE
Link to the notebook : https://github.com/bhattbhavesh91/tflite-tutorials/blob/master/tflite-part-2.ipynb
You can quantize an already-trained float TensorFlow model when you convert it to TensorFlow Lite format using the TensorFlow Lite Converter.
If you do have any questions with what we covered in this video then feel free to ask in the comment section below & I'll do my best to answer those.
If you enjoy these tutorials & would like to support them then the easiest way is to simply like the video & give it a thumbs up & also it's a huge help to share these videos with anyone who you think would find them useful.
Please consider clicking the SUBSCRIBE button to be notified for future videos & thank you all for watching.
You can find me on:
Blog - http://bhattbhavesh91.github.io
Twitter - https://twitter.com/_bhaveshbhatt
GitHub - https://github.com/bhattbhavesh91
Medium - https://medium.com/@bhattbhavesh91
#tflite #Quantiziation
Видео Post-training Quantization in TensorFlow Lite (TFLite) канала Bhavesh Bhatt
Показать
Комментарии отсутствуют
Информация о видео
Другие видео канала
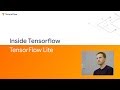
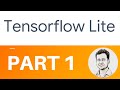
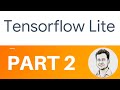
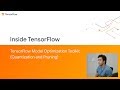
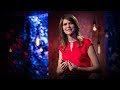
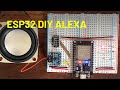

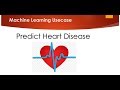
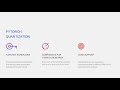


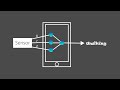
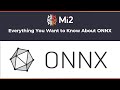
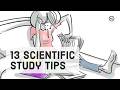
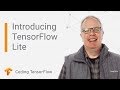
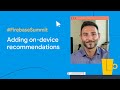
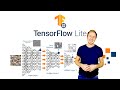
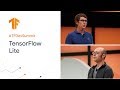
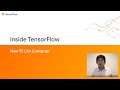
