NÜWA: Visual Synthesis Pre-training for Neural visUal World creAtion (ML Research Paper Explained)
#nuwa #microsoft #generative
NÜWA is a unifying architecture that can ingest text, images, and videos and brings all of them into a quantized latent representation to support a multitude of visual generation tasks, such as text-to-image, text-guided video manipulation, or sketch-to-video. This paper details how the encoders for the different modalities are constructed, and how the latent representation is transformed using their novel 3D nearby self-attention layers. Experiments are shown on 8 different visual generation tasks that the model supports.
OUTLINE:
0:00 - Intro & Outline
1:20 - Sponsor: ClearML
3:35 - Tasks & Naming
5:10 - The problem with recurrent image generation
7:35 - Creating a shared latent space w/ Vector Quantization
23:20 - Transforming the latent representation
26:25 - Recap: Self- and Cross-Attention
28:50 - 3D Nearby Self-Attention
41:20 - Pre-Training Objective
46:05 - Experimental Results
50:40 - Conclusion & Comments
Paper: https://arxiv.org/abs/2111.12417
Github: https://github.com/microsoft/NUWA
Sponsor: ClearML
https://clear.ml
Abstract:
This paper presents a unified multimodal pre-trained model called NÜWA that can generate new or manipulate existing visual data (i.e., images and videos) for various visual synthesis tasks. To cover language, image, and video at the same time for different scenarios, a 3D transformer encoder-decoder framework is designed, which can not only deal with videos as 3D data but also adapt to texts and images as 1D and 2D data, respectively. A 3D Nearby Attention (3DNA) mechanism is also proposed to consider the nature of the visual data and reduce the computational complexity. We evaluate NÜWA on 8 downstream tasks. Compared to several strong baselines, NÜWA achieves state-of-the-art results on text-to-image generation, text-to-video generation, video prediction, etc. Furthermore, it also shows surprisingly good zero-shot capabilities on text-guided image and video manipulation tasks. Project repo is this https URL.
Authors: Chenfei Wu, Jian Liang, Lei Ji, Fan Yang, Yuejian Fang, Daxin Jiang, Nan Duan
Links:
TabNine Code Completion (Referral): http://bit.ly/tabnine-yannick
YouTube: https://www.youtube.com/c/yannickilcher
Twitter: https://twitter.com/ykilcher
Discord: https://discord.gg/4H8xxDF
BitChute: https://www.bitchute.com/channel/yannic-kilcher
LinkedIn: https://www.linkedin.com/in/ykilcher
BiliBili: https://space.bilibili.com/2017636191
If you want to support me, the best thing to do is to share out the content :)
If you want to support me financially (completely optional and voluntary, but a lot of people have asked for this):
SubscribeStar: https://www.subscribestar.com/yannickilcher
Patreon: https://www.patreon.com/yannickilcher
Bitcoin (BTC): bc1q49lsw3q325tr58ygf8sudx2dqfguclvngvy2cq
Ethereum (ETH): 0x7ad3513E3B8f66799f507Aa7874b1B0eBC7F85e2
Litecoin (LTC): LQW2TRyKYetVC8WjFkhpPhtpbDM4Vw7r9m
Monero (XMR): 4ACL8AGrEo5hAir8A9CeVrW8pEauWvnp1WnSDZxW7tziCDLhZAGsgzhRQABDnFy8yuM9fWJDviJPHKRjV4FWt19CJZN9D4n
Видео NÜWA: Visual Synthesis Pre-training for Neural visUal World creAtion (ML Research Paper Explained) канала Yannic Kilcher
NÜWA is a unifying architecture that can ingest text, images, and videos and brings all of them into a quantized latent representation to support a multitude of visual generation tasks, such as text-to-image, text-guided video manipulation, or sketch-to-video. This paper details how the encoders for the different modalities are constructed, and how the latent representation is transformed using their novel 3D nearby self-attention layers. Experiments are shown on 8 different visual generation tasks that the model supports.
OUTLINE:
0:00 - Intro & Outline
1:20 - Sponsor: ClearML
3:35 - Tasks & Naming
5:10 - The problem with recurrent image generation
7:35 - Creating a shared latent space w/ Vector Quantization
23:20 - Transforming the latent representation
26:25 - Recap: Self- and Cross-Attention
28:50 - 3D Nearby Self-Attention
41:20 - Pre-Training Objective
46:05 - Experimental Results
50:40 - Conclusion & Comments
Paper: https://arxiv.org/abs/2111.12417
Github: https://github.com/microsoft/NUWA
Sponsor: ClearML
https://clear.ml
Abstract:
This paper presents a unified multimodal pre-trained model called NÜWA that can generate new or manipulate existing visual data (i.e., images and videos) for various visual synthesis tasks. To cover language, image, and video at the same time for different scenarios, a 3D transformer encoder-decoder framework is designed, which can not only deal with videos as 3D data but also adapt to texts and images as 1D and 2D data, respectively. A 3D Nearby Attention (3DNA) mechanism is also proposed to consider the nature of the visual data and reduce the computational complexity. We evaluate NÜWA on 8 downstream tasks. Compared to several strong baselines, NÜWA achieves state-of-the-art results on text-to-image generation, text-to-video generation, video prediction, etc. Furthermore, it also shows surprisingly good zero-shot capabilities on text-guided image and video manipulation tasks. Project repo is this https URL.
Authors: Chenfei Wu, Jian Liang, Lei Ji, Fan Yang, Yuejian Fang, Daxin Jiang, Nan Duan
Links:
TabNine Code Completion (Referral): http://bit.ly/tabnine-yannick
YouTube: https://www.youtube.com/c/yannickilcher
Twitter: https://twitter.com/ykilcher
Discord: https://discord.gg/4H8xxDF
BitChute: https://www.bitchute.com/channel/yannic-kilcher
LinkedIn: https://www.linkedin.com/in/ykilcher
BiliBili: https://space.bilibili.com/2017636191
If you want to support me, the best thing to do is to share out the content :)
If you want to support me financially (completely optional and voluntary, but a lot of people have asked for this):
SubscribeStar: https://www.subscribestar.com/yannickilcher
Patreon: https://www.patreon.com/yannickilcher
Bitcoin (BTC): bc1q49lsw3q325tr58ygf8sudx2dqfguclvngvy2cq
Ethereum (ETH): 0x7ad3513E3B8f66799f507Aa7874b1B0eBC7F85e2
Litecoin (LTC): LQW2TRyKYetVC8WjFkhpPhtpbDM4Vw7r9m
Monero (XMR): 4ACL8AGrEo5hAir8A9CeVrW8pEauWvnp1WnSDZxW7tziCDLhZAGsgzhRQABDnFy8yuM9fWJDviJPHKRjV4FWt19CJZN9D4n
Видео NÜWA: Visual Synthesis Pre-training for Neural visUal World creAtion (ML Research Paper Explained) канала Yannic Kilcher
Показать
Комментарии отсутствуют
Информация о видео
Другие видео канала
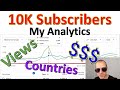
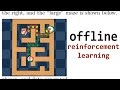
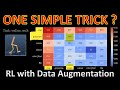
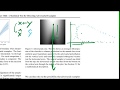
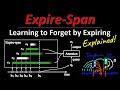
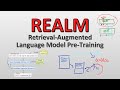
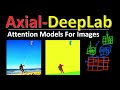
![[Classic] Playing Atari with Deep Reinforcement Learning (Paper Explained)](https://i.ytimg.com/vi/rFwQDDbYTm4/default.jpg)
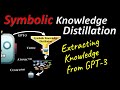
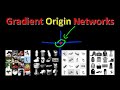
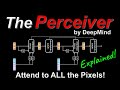
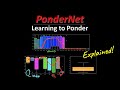
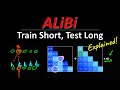
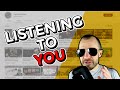
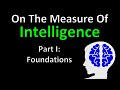
![[ML News] Uber: Deep Learning for ETA | MuZero Video Compression | Block-NeRF | EfficientNet-X](https://i.ytimg.com/vi/fEKZC9mta8w/default.jpg)
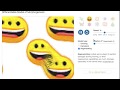
![[ML News] DeepMind's Flamingo Image-Text model | Locked-Image Tuning | Jurassic X & MRKL](https://i.ytimg.com/vi/smUHQndcmOY/default.jpg)
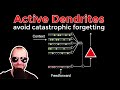
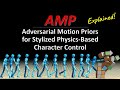
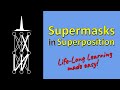