The biggest problem in AI? Machines have no common sense. | Gary Marcus | Big Think
The biggest problem in AI? Machines have no common sense
New videos DAILY: https://bigth.ink
Join Big Think Edge for exclusive video lessons from top thinkers and doers: https://bigth.ink/Edge
----------------------------------------------------------------------------------
There are a lot of people in the tech world who think that if we collect as much data as much, and run a lot of statistics, that we will be able to develop robots where artificial "intelligence" organically emerges.
However, many A.I.'s that currently exist aren't close to being "intelligent," it's difficult to even program common sense into them. The reason for this is because correlation doesn't always equal causation — robots that operate on correlation alone may have skewed algorithms in which to operate in the real world.
When it comes to performing simple tasks, such as opening a door, we currently don't know how to encode that information — the varied process that is sometimes required in differing situations, i.e. jiggling the key, turning the key just right — into a language that a computer can understand.
----------------------------------------------------------------------------------
GARY MARCUS
Dr. Gary Marcus is the director of the NYU Infant Language Learning Center, and a professor of psychology at New York University. He is the author of "The Birth of the Mind," "The Algebraic Mind: Integrating Connectionism and Cognitive Science," and "Kluge: The Haphazard Construction of the Human Mind." Marcus's research on developmental cognitive neuroscience has been published in over forty articles in leading journals, and in 1996 he won the Robert L. Fantz award for new investigators in cognitive development.
Marcus contributed an idea to Big Think's "Dangerous Ideas" blog, suggesting that we should develop Google-like chips to implant in our brains and enhance our memory.
----------------------------------------------------------------------------------
TRANSCRIPT:
GARY MARCUS: The dominant vision in the field right now is, collect a lot of data, run a lot of statistics, and intelligence will emerge. And I think that's wrong. I think that having a lot of data is important, and collecting a lot of statistics is important. But I think what we also need is deep understanding, not just so-called "deep learning." So deep learning finds what's typically correlated, but we all know that correlation is not the same thing as causation. And even though we all know that and everybody learned that in Intro to Psych, or should have learned that in Intro to Psych they should have learned that you don't know whether cigarettes cause smoking just from the statistics we have. We have to make causal inferences and do careful studies. We all know that causation and correlation are not the same thing.
Have right now as AIs-- giant correlation machines. And it works, if you have enough control of the data relative to the problem that you're studying that you can exhaust the problem, to beat the problem into submission. So you can do that with go. You could play this game, over and over again, the rules never change. They haven't changed in 2,000 years. And the board is always the same size. And so you can just get enough statistics about what tends to work, and you're good to go. But if you want to use the same techniques for natural language understanding, for example, or to guide a domestic robot through your house, it's not going to work. So the domestic robot in your house is going to keep seeing new situations. And your natural language understanding system, every dialogue is going to be different. It's not really going to work. So yeah, you can talk to Alexa and you can say, please turn on my over and over again, get statistics on that. It's fine. But there's no machine in the world T=that we're having right now. It's just not anywhere near a reality and you're not going to be able to do it with the statistics, because there's not enough similar stuff going on.
Probably the single best thing that we could do to make our machines smarter is to give them common sense, which is much harder than it sounds like. I mean, first you might say, what is common sense? And what we settled on in the book that we wrote is that common sense is the knowledge that's commonly held. that ordinary people have. And yet machines don't. So machines are really good at things like, I don't know, converting metrics -- you know, converting from the English system to the metric system. Things that are nice, and precise, and factual, and easily stated. But things that are a little bit less sharply stated, like how you open a door, machines don't understand the first thing. So there's actually a competition right now for opening doors. So if somebody uploaded data sets from 500 different doors, and they're hoping that...
For the full transcript, check out: https://bigthink.com/videos/machines-have-no-common-sense-
Видео The biggest problem in AI? Machines have no common sense. | Gary Marcus | Big Think канала Big Think
New videos DAILY: https://bigth.ink
Join Big Think Edge for exclusive video lessons from top thinkers and doers: https://bigth.ink/Edge
----------------------------------------------------------------------------------
There are a lot of people in the tech world who think that if we collect as much data as much, and run a lot of statistics, that we will be able to develop robots where artificial "intelligence" organically emerges.
However, many A.I.'s that currently exist aren't close to being "intelligent," it's difficult to even program common sense into them. The reason for this is because correlation doesn't always equal causation — robots that operate on correlation alone may have skewed algorithms in which to operate in the real world.
When it comes to performing simple tasks, such as opening a door, we currently don't know how to encode that information — the varied process that is sometimes required in differing situations, i.e. jiggling the key, turning the key just right — into a language that a computer can understand.
----------------------------------------------------------------------------------
GARY MARCUS
Dr. Gary Marcus is the director of the NYU Infant Language Learning Center, and a professor of psychology at New York University. He is the author of "The Birth of the Mind," "The Algebraic Mind: Integrating Connectionism and Cognitive Science," and "Kluge: The Haphazard Construction of the Human Mind." Marcus's research on developmental cognitive neuroscience has been published in over forty articles in leading journals, and in 1996 he won the Robert L. Fantz award for new investigators in cognitive development.
Marcus contributed an idea to Big Think's "Dangerous Ideas" blog, suggesting that we should develop Google-like chips to implant in our brains and enhance our memory.
----------------------------------------------------------------------------------
TRANSCRIPT:
GARY MARCUS: The dominant vision in the field right now is, collect a lot of data, run a lot of statistics, and intelligence will emerge. And I think that's wrong. I think that having a lot of data is important, and collecting a lot of statistics is important. But I think what we also need is deep understanding, not just so-called "deep learning." So deep learning finds what's typically correlated, but we all know that correlation is not the same thing as causation. And even though we all know that and everybody learned that in Intro to Psych, or should have learned that in Intro to Psych they should have learned that you don't know whether cigarettes cause smoking just from the statistics we have. We have to make causal inferences and do careful studies. We all know that causation and correlation are not the same thing.
Have right now as AIs-- giant correlation machines. And it works, if you have enough control of the data relative to the problem that you're studying that you can exhaust the problem, to beat the problem into submission. So you can do that with go. You could play this game, over and over again, the rules never change. They haven't changed in 2,000 years. And the board is always the same size. And so you can just get enough statistics about what tends to work, and you're good to go. But if you want to use the same techniques for natural language understanding, for example, or to guide a domestic robot through your house, it's not going to work. So the domestic robot in your house is going to keep seeing new situations. And your natural language understanding system, every dialogue is going to be different. It's not really going to work. So yeah, you can talk to Alexa and you can say, please turn on my over and over again, get statistics on that. It's fine. But there's no machine in the world T=that we're having right now. It's just not anywhere near a reality and you're not going to be able to do it with the statistics, because there's not enough similar stuff going on.
Probably the single best thing that we could do to make our machines smarter is to give them common sense, which is much harder than it sounds like. I mean, first you might say, what is common sense? And what we settled on in the book that we wrote is that common sense is the knowledge that's commonly held. that ordinary people have. And yet machines don't. So machines are really good at things like, I don't know, converting metrics -- you know, converting from the English system to the metric system. Things that are nice, and precise, and factual, and easily stated. But things that are a little bit less sharply stated, like how you open a door, machines don't understand the first thing. So there's actually a competition right now for opening doors. So if somebody uploaded data sets from 500 different doors, and they're hoping that...
For the full transcript, check out: https://bigthink.com/videos/machines-have-no-common-sense-
Видео The biggest problem in AI? Machines have no common sense. | Gary Marcus | Big Think канала Big Think
Показать
Комментарии отсутствуют
Информация о видео
Другие видео канала
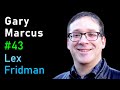
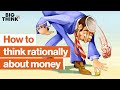
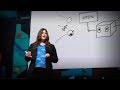
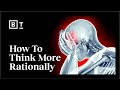
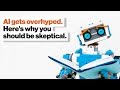
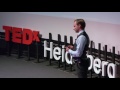
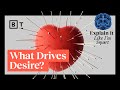
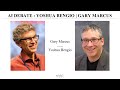

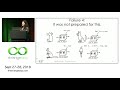
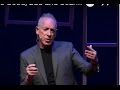
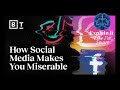

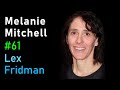
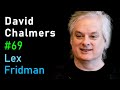
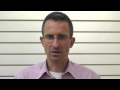
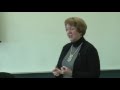
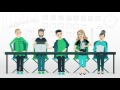
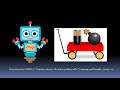
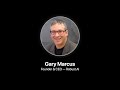