What is the Statistical Complexity of Reinforcement Learning?
Sham Kakade (Harvard and MSR)
https://simons.berkeley.edu/talks/what-statistical-complexity-reinforcement-learning
Multi-Agent Reinforcement Learning and Bandit Learning
A fundamental question in the theory of reinforcement learning is what (representational or structural) conditions govern our ability to generalize and avoid the curse of dimensionality. With regards to supervised learning, these questions are well understood theoretically: practically, we have overwhelming evidence on the value of representational learning (say through modern deep networks) as a means for sample efficient learning, and, theoretically, there are well-known complexity measures (e.g. the VC dimension and Rademacher complexity) that govern the statistical complexity of learning. Providing an analogous theory for reinforcement learning is far more challenging, where even characterizing any structural conditions which support sample efficient generalization is far less well understood. This talk will highlight recent advances towards characterizing when generalization is possible in reinforcement learning (both in online and offline settings), focusing on both necessary and sufficient conditions. In particular, we will introduce a new complexity measure, the Decision-Estimation Coefficient, that is proven to be necessary (and, essentially, sufficient) for sample-efficient interactive learning.
Видео What is the Statistical Complexity of Reinforcement Learning? канала Simons Institute
https://simons.berkeley.edu/talks/what-statistical-complexity-reinforcement-learning
Multi-Agent Reinforcement Learning and Bandit Learning
A fundamental question in the theory of reinforcement learning is what (representational or structural) conditions govern our ability to generalize and avoid the curse of dimensionality. With regards to supervised learning, these questions are well understood theoretically: practically, we have overwhelming evidence on the value of representational learning (say through modern deep networks) as a means for sample efficient learning, and, theoretically, there are well-known complexity measures (e.g. the VC dimension and Rademacher complexity) that govern the statistical complexity of learning. Providing an analogous theory for reinforcement learning is far more challenging, where even characterizing any structural conditions which support sample efficient generalization is far less well understood. This talk will highlight recent advances towards characterizing when generalization is possible in reinforcement learning (both in online and offline settings), focusing on both necessary and sufficient conditions. In particular, we will introduce a new complexity measure, the Decision-Estimation Coefficient, that is proven to be necessary (and, essentially, sufficient) for sample-efficient interactive learning.
Видео What is the Statistical Complexity of Reinforcement Learning? канала Simons Institute
Показать
Комментарии отсутствуют
Информация о видео
Другие видео канала
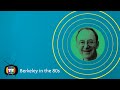
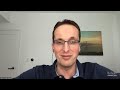
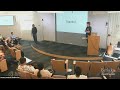
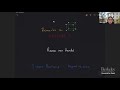
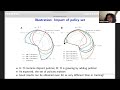
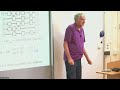
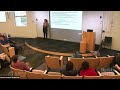
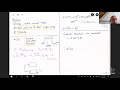
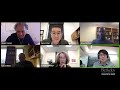
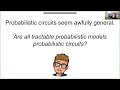
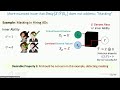
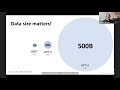
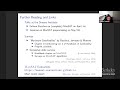
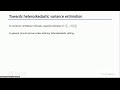
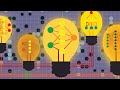
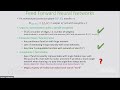
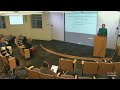
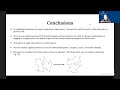
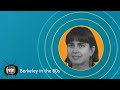
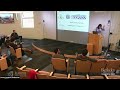
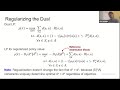