Animation: Variational Autoencoder
A variational autoencoder is a type of neural network that learns to compress (encode) data, in such a way that one can later randomly sample data.
Here we show training of a variational autoencoder on a data set of wave packets that have been placed with a random location and amplitude. Upper left: test set, with VAE decoder results shown in orange; upper right: latent space distribution, with color coding for actual location (left) or amplitude (right) of any data point; lower right: latent space distributions for the few samples from the test set (in each case, the Gaussian spread in latent space is also indicated); lower left: loss evolution.
2021 by Florian Marquardt. For the full explanation, watch https://www.youtube.com/watch?v=bSta4s439-I .
This animation is part of the online lecture series "Advanced Machine Learning for Physics, Science, and Artificial Scientific Discovery". See the website https://pad.gwdg.de/s/2021_AdvancedMachineLearningForScience# and the channel with the full lecture videos: https://www.youtube.com/playlist?list=PLemsnf33Vij4-kv-JTjDthaGUYUnQbbws.
Видео Animation: Variational Autoencoder канала Florian Marquardt
Here we show training of a variational autoencoder on a data set of wave packets that have been placed with a random location and amplitude. Upper left: test set, with VAE decoder results shown in orange; upper right: latent space distribution, with color coding for actual location (left) or amplitude (right) of any data point; lower right: latent space distributions for the few samples from the test set (in each case, the Gaussian spread in latent space is also indicated); lower left: loss evolution.
2021 by Florian Marquardt. For the full explanation, watch https://www.youtube.com/watch?v=bSta4s439-I .
This animation is part of the online lecture series "Advanced Machine Learning for Physics, Science, and Artificial Scientific Discovery". See the website https://pad.gwdg.de/s/2021_AdvancedMachineLearningForScience# and the channel with the full lecture videos: https://www.youtube.com/playlist?list=PLemsnf33Vij4-kv-JTjDthaGUYUnQbbws.
Видео Animation: Variational Autoencoder канала Florian Marquardt
Показать
Комментарии отсутствуют
Информация о видео
Другие видео канала
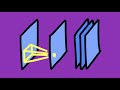

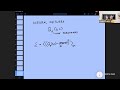
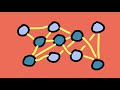
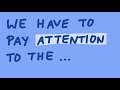
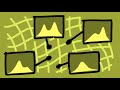
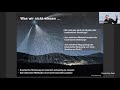
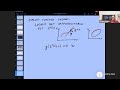
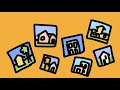
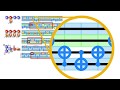
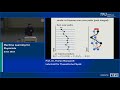
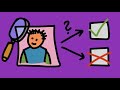
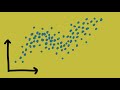
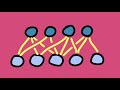
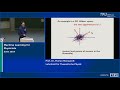
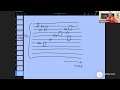
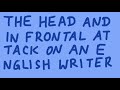
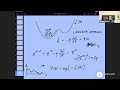
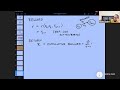
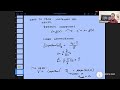