Monocular Real-time Hand Shape and Motion Capture using Multi-modal Data - CVPR 2020
Abstract
We present a novel method for monocular hand shape and pose estimation at unprecedented runtime performance of 100fps and at state-of-the-art accuracy. This is enabled by a new learning based architecture designed such that it can make use of all the sources of available hand training data: image data with either 2D or 3D annotations, as well as stand-alone 3D animations without corresponding image data. It features a 3D hand joint detection module and an inverse kinematics module which regresses not only 3D joint positions but also maps them to joint rotations in a single feed-forward pass. This output makes the method more directly usable for applications in computer vision and graphics compared to only regressing 3D joint positions. We demonstrate that our architectural design leads to a significant quantitative and qualitative improvement over the state of the art on several challenging benchmarks.
Y. Zhou, M. Habermann, W. Xu, V. Golyanik, C. Theobalt and F. Xu
Monocular Real-time Hand Shape and Motion Capture using Multi-modal Data, Proc. of IEEE Conf. on Computer Vision and Pattern Recognition (CVPR), 2020
Видео Monocular Real-time Hand Shape and Motion Capture using Multi-modal Data - CVPR 2020 канала Christian Theobalt
We present a novel method for monocular hand shape and pose estimation at unprecedented runtime performance of 100fps and at state-of-the-art accuracy. This is enabled by a new learning based architecture designed such that it can make use of all the sources of available hand training data: image data with either 2D or 3D annotations, as well as stand-alone 3D animations without corresponding image data. It features a 3D hand joint detection module and an inverse kinematics module which regresses not only 3D joint positions but also maps them to joint rotations in a single feed-forward pass. This output makes the method more directly usable for applications in computer vision and graphics compared to only regressing 3D joint positions. We demonstrate that our architectural design leads to a significant quantitative and qualitative improvement over the state of the art on several challenging benchmarks.
Y. Zhou, M. Habermann, W. Xu, V. Golyanik, C. Theobalt and F. Xu
Monocular Real-time Hand Shape and Motion Capture using Multi-modal Data, Proc. of IEEE Conf. on Computer Vision and Pattern Recognition (CVPR), 2020
Видео Monocular Real-time Hand Shape and Motion Capture using Multi-modal Data - CVPR 2020 канала Christian Theobalt
Показать
Комментарии отсутствуют
Информация о видео
Другие видео канала
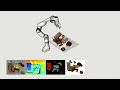
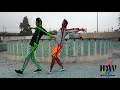
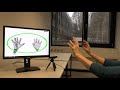
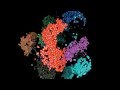
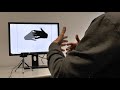
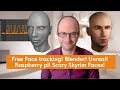

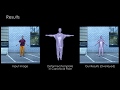
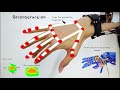
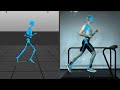
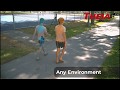
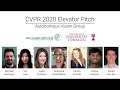
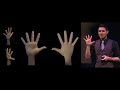

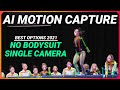
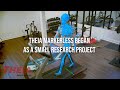
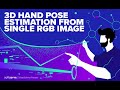
![[Talk] Analyzing CNN Artifacts in Discriminative and Generative Models (02/20)](https://i.ytimg.com/vi/aNDwHRxWTa0/default.jpg)
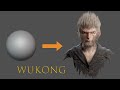
