Bridging the Gap Between Anchor-Based and Anchor-Free Detection via Adaptive Training Sample Sele...
Authors: Shifeng Zhang, Cheng Chi, Yongqiang Yao, Zhen Lei, Stan Z. Li Description: Object detection has been dominated by anchor-based detectors for several years. Recently, anchor-free detectors have become popular due to the proposal of FPN and Focal Loss. In this paper, we first point out that the essential difference between anchor-based and anchor-free detection is actually how to define positive and negative training samples, which leads to the performance gap between them. If they adopt the same definition of positive and negative samples during training, there is no obvious difference in the final performance, no matter regressing from a box or a point. This shows that how to select positive and negative training samples is important for current object detectors. Then, we propose an Adaptive Training Sample Selection (ATSS) to automatically select positive and negative samples according to statistical characteristics of object. It significantly improves the performance of anchor-based and anchor-free detectors and bridges the gap between them. Finally, we discuss the necessity of tiling multiple anchors per location on the image to detect objects. Extensive experiments conducted on MS COCO support our aforementioned analysis and conclusions. With the newly introduced ATSS, we improve state-of-the-art detectors by a large margin to 50.7% AP without introducing any overhead. The code is available at https://github.com/sfzhang15/ATSS.
Видео Bridging the Gap Between Anchor-Based and Anchor-Free Detection via Adaptive Training Sample Sele... канала ComputerVisionFoundation Videos
Видео Bridging the Gap Between Anchor-Based and Anchor-Free Detection via Adaptive Training Sample Sele... канала ComputerVisionFoundation Videos
Показать
Комментарии отсутствуют
Информация о видео
21 июля 2020 г. 2:56:42
00:05:01
Другие видео канала
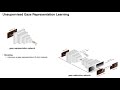
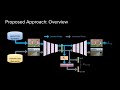
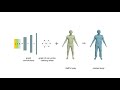
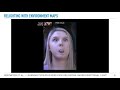
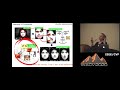
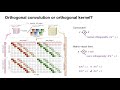
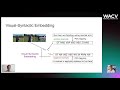

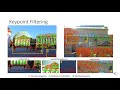

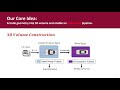
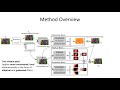
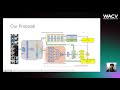
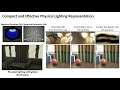
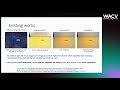
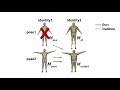
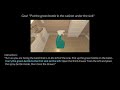
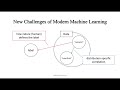
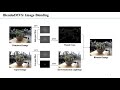
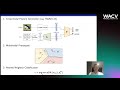
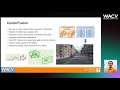