Differentiable Physics (for Deep Learning), Overview Talk by Nils Thuerey
In this talk Nils explains recent research works that shows how to employ differentiable PDE solvers for deep learning. A central aim here is to improve the outcomes of numerical simulations. Results for reducing the numerical error in iterative solvers, and long-term control of physical systems are demonstrated. Source code is available at: https://github.com/tum-pbs/PhiFlow
The two research papers discussed in the talk can be found at:
https://ge.in.tum.de/publications/2020-um-solver-in-the-loop/ & https://arxiv.org/pdf/2007.00016
https://ge.in.tum.de/publications/2020-iclr-holl/ & https://arxiv.org/pdf/2001.07457
Addendum: the missing video at 33:45 is https://ge.in.tum.de/download/2020-iclr-holl/supplemental/Fig5b%20shapes%202%20refined/sequence_0.mp4 , it shows a much more "intuitive" solution compared to the single-case optimization.
Видео Differentiable Physics (for Deep Learning), Overview Talk by Nils Thuerey канала Nils Thuerey
The two research papers discussed in the talk can be found at:
https://ge.in.tum.de/publications/2020-um-solver-in-the-loop/ & https://arxiv.org/pdf/2007.00016
https://ge.in.tum.de/publications/2020-iclr-holl/ & https://arxiv.org/pdf/2001.07457
Addendum: the missing video at 33:45 is https://ge.in.tum.de/download/2020-iclr-holl/supplemental/Fig5b%20shapes%202%20refined/sequence_0.mp4 , it shows a much more "intuitive" solution compared to the single-case optimization.
Видео Differentiable Physics (for Deep Learning), Overview Talk by Nils Thuerey канала Nils Thuerey
Показать
Комментарии отсутствуют
Информация о видео
Другие видео канала
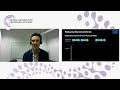

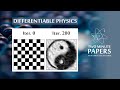


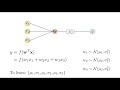
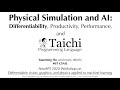
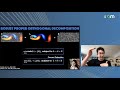
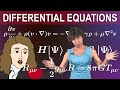
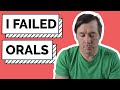
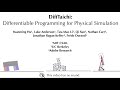
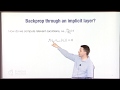
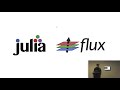
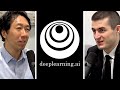
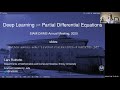
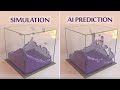
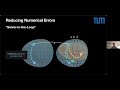
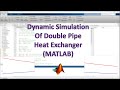
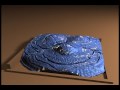
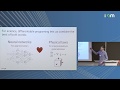