Anima Anandkumar - Neural operator: A new paradigm for learning PDEs
Talk starts at 1:50
Prof. Anima Anandkumar from Caltech/NVIDIA speaking in the Data-Driven Methods for Science and Engineering Seminar on March 5, 2021
For more information including past and upcoming talks, visit: http://www.databookuw.com/seminars/
Sign up for notifications of future talks: https://mailman.u.washington.edu/mailman/listinfo/datadriven-seminar
Abstract: Partial Differential Equations (PDE) lay the foundation for modeling a wide variety of scientific phenomena. Traditional solvers tend to be slow when high-fidelity solutions are needed. We introduce neural-operator, a data-driven approach that aims to directly learn the solution operator of PDEs. Unlike neural networks that learn function mapping between finite-dimensional spaces, neural operator extends that to learning operator between infinite-dimensional spaces. This makes the neural operator independent of resolution and grid of training data and allows for zero-shot generalization to higher resolution evaluations. We find that the neural operator is able to solve the Navier-Stokes equation in the turbulent regime with a 1000x speedup compared to traditional methods.
http://tensorlab.cms.caltech.edu/users/anima/
Видео Anima Anandkumar - Neural operator: A new paradigm for learning PDEs канала Physics Informed Machine Learning
Prof. Anima Anandkumar from Caltech/NVIDIA speaking in the Data-Driven Methods for Science and Engineering Seminar on March 5, 2021
For more information including past and upcoming talks, visit: http://www.databookuw.com/seminars/
Sign up for notifications of future talks: https://mailman.u.washington.edu/mailman/listinfo/datadriven-seminar
Abstract: Partial Differential Equations (PDE) lay the foundation for modeling a wide variety of scientific phenomena. Traditional solvers tend to be slow when high-fidelity solutions are needed. We introduce neural-operator, a data-driven approach that aims to directly learn the solution operator of PDEs. Unlike neural networks that learn function mapping between finite-dimensional spaces, neural operator extends that to learning operator between infinite-dimensional spaces. This makes the neural operator independent of resolution and grid of training data and allows for zero-shot generalization to higher resolution evaluations. We find that the neural operator is able to solve the Navier-Stokes equation in the turbulent regime with a 1000x speedup compared to traditional methods.
http://tensorlab.cms.caltech.edu/users/anima/
Видео Anima Anandkumar - Neural operator: A new paradigm for learning PDEs канала Physics Informed Machine Learning
Показать
Комментарии отсутствуют
Информация о видео
6 марта 2021 г. 0:46:32
00:59:56
Другие видео канала
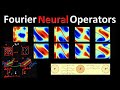
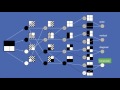
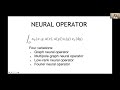
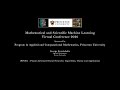
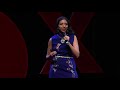

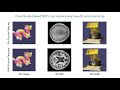
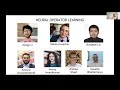
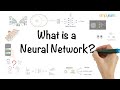
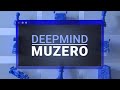
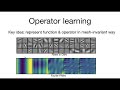
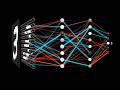
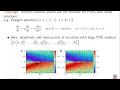
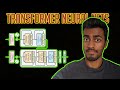
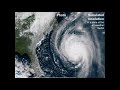
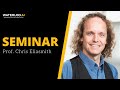
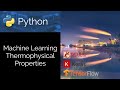
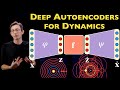
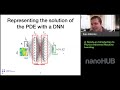
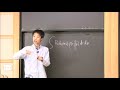