Understanding Approximate Inference in Bayesian Neural Networks: A Joint Talk
Do we need rich posterior approximations in variational inference?
Mean-field variational inference and Monte Carlo dropout are both widely used variational approximations for Bayesian deep learning. But how big a price do we pay for the restrictions these approximations impose? Two recent papers at NeurIPS have evaluated this question both theoretically and empirically.
Although both papers address the same topics, their emphases and conclusions on the danger/potential of approximate inference in shallow and deep networks are somewhat different. This informal event will be an opportunity to discuss these matters in more detail.
The authors of both papers will each give a short talk on Zoom, followed by half an hour of discussion and audience questions, moderated by Dr. Yingzhen Li (Imperial College).
Recorded:
Date: Thursday 11 March 2021
Time: 17:00 GMT
"On the Expressiveness of Approximate Inference in Bayesian Neural Networks". Andrew Foong, David Burt, Yingzhen Li, Richard Turner, NeurIPS 2020 [https://arxiv.org/abs/1909.00719]
Slides: https://sebastianfarquhar.com/deepmeanfield/joint_talk_handout.pdf
"Liberty or Depth: Deep Bayesian Neural Nets Do Not Need Complex Weight Posterior Approximations". Sebastian Farquhar, Lewis Smith, Yarin Gal, NeurIPS 2020 [https://arxiv.org/abs/2002.03704]
Slides: https://sebastianfarquhar.com/deepmeanfield/joint_talk_liberty.pdf
Видео Understanding Approximate Inference in Bayesian Neural Networks: A Joint Talk канала OATML research group
Mean-field variational inference and Monte Carlo dropout are both widely used variational approximations for Bayesian deep learning. But how big a price do we pay for the restrictions these approximations impose? Two recent papers at NeurIPS have evaluated this question both theoretically and empirically.
Although both papers address the same topics, their emphases and conclusions on the danger/potential of approximate inference in shallow and deep networks are somewhat different. This informal event will be an opportunity to discuss these matters in more detail.
The authors of both papers will each give a short talk on Zoom, followed by half an hour of discussion and audience questions, moderated by Dr. Yingzhen Li (Imperial College).
Recorded:
Date: Thursday 11 March 2021
Time: 17:00 GMT
"On the Expressiveness of Approximate Inference in Bayesian Neural Networks". Andrew Foong, David Burt, Yingzhen Li, Richard Turner, NeurIPS 2020 [https://arxiv.org/abs/1909.00719]
Slides: https://sebastianfarquhar.com/deepmeanfield/joint_talk_handout.pdf
"Liberty or Depth: Deep Bayesian Neural Nets Do Not Need Complex Weight Posterior Approximations". Sebastian Farquhar, Lewis Smith, Yarin Gal, NeurIPS 2020 [https://arxiv.org/abs/2002.03704]
Slides: https://sebastianfarquhar.com/deepmeanfield/joint_talk_liberty.pdf
Видео Understanding Approximate Inference in Bayesian Neural Networks: A Joint Talk канала OATML research group
Показать
Комментарии отсутствуют
Информация о видео
Другие видео канала
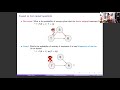
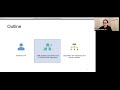
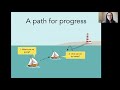
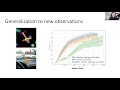
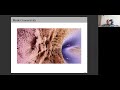
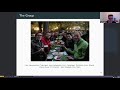