Lecture 15.3 — Deep autoencoders for document retrieval [Neural Networks for Machine Learning]
Lecture from the course Neural Networks for Machine Learning, as taught by Geoffrey Hinton (University of Toronto) on Coursera in 2012.
Link to the course (login required): https://class.coursera.org/neuralnets-2012-001
Видео Lecture 15.3 — Deep autoencoders for document retrieval [Neural Networks for Machine Learning] канала Colin Reckons
Link to the course (login required): https://class.coursera.org/neuralnets-2012-001
Видео Lecture 15.3 — Deep autoencoders for document retrieval [Neural Networks for Machine Learning] канала Colin Reckons
Показать
Комментарии отсутствуют
Информация о видео
Другие видео канала
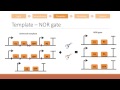
![Lecture 5.2 — Achieving viewpoint invariance [Neural Networks for Machine Learning]](https://i.ytimg.com/vi/SxD-YVxIygc/default.jpg)
![Lecture 14.5 — RBMs are infinite sigmoid belief nets [Neural Networks for Machine Learning]](https://i.ytimg.com/vi/evnofrn-QHo/default.jpg)
![10.1 — Why it helps to combine models [Neural Networks for Machine Learning]](https://i.ytimg.com/vi/yIIFnTkvhrQ/default.jpg)
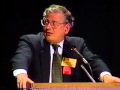

![Lecture 7.3 — A toy example of training an RNN [Neural Networks for Machine Learning]](https://i.ytimg.com/vi/bVGdxHgxG34/default.jpg)
![Lecture 8.3 — Predicting the next character using HF [Neural Networks for Machine Learning]](https://i.ytimg.com/vi/bNcb4MhSZ0s/default.jpg)
![Lecture 16.2 — Hierarchical Coordinate Frames [Neural Networks for Machine Learning]](https://i.ytimg.com/vi/z0qPxIf4l9I/default.jpg)
![Lecture 13.3 — Learning sigmoid belief nets [Neural Networks for Machine Learning]](https://i.ytimg.com/vi/VxfSqmxFl1c/default.jpg)
![Lecture 3.5 — Using the derivatives from backpropagation [Neural Networks for Machine Learning]](https://i.ytimg.com/vi/vlSI79ringA/default.jpg)
![Lecture 4.4 — Neuro-probabilistic language models [Neural Networks for Machine Learning]](https://i.ytimg.com/vi/_1zwTzpc50Q/default.jpg)
![Lecture 15.5 — Learning binary codes for image retrieval [Neural Networks for Machine Learning]](https://i.ytimg.com/vi/MSYmyJgYOnU/default.jpg)
![Lecture 13.2 — Belief Nets [Neural Networks for Machine Learning]](https://i.ytimg.com/vi/6yxJeySclIc/default.jpg)
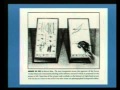
![Lecture 5.1 — Why object recognition is difficult [Neural Networks for Machine Learning]](https://i.ytimg.com/vi/Qx3i7VWYwhI/default.jpg)
![Lecture 15.4 — Semantic Hashing [Neural Networks for Machine Learning]](https://i.ytimg.com/vi/swjncYpcLsk/default.jpg)
![Lecture 15.6 — Shallow autoencoders for pre-training [Neural Networks for Machine Learning]](https://i.ytimg.com/vi/e_n2hht9Yc8/default.jpg)
![Lecture 9.1 — Overview of ways to improve generalization [Neural Networks for Machine Learning]](https://i.ytimg.com/vi/W0SP8FTmGW0/default.jpg)
![Lecture 9.5 — The Bayesian interpretation of weight decay [Neural Networks for Machine Learning]](https://i.ytimg.com/vi/vEPQNwxd1Y4/default.jpg)
![Lecture 11.4 — Using stochastic units to improve search [Neural Networks for Machine Learning]](https://i.ytimg.com/vi/hmL9Kozwf7A/default.jpg)