Automatic Differentiation of Damaged and Unharmed Grapes Using RGB Images and CNNs
Presentation given by Laura Zabawa at the ECCV 2020 Workshop on
Computer Vision Problems in Plant Phenotyping (CVPPP 2020)
Видео Automatic Differentiation of Damaged and Unharmed Grapes Using RGB Images and CNNs канала Ribana Roscher
Computer Vision Problems in Plant Phenotyping (CVPPP 2020)
Видео Automatic Differentiation of Damaged and Unharmed Grapes Using RGB Images and CNNs канала Ribana Roscher
Показать
Комментарии отсутствуют
Информация о видео
Другие видео канала
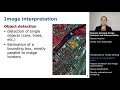
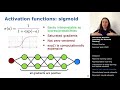
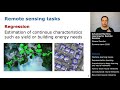
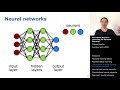
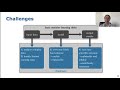


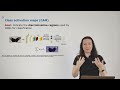
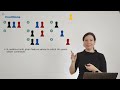
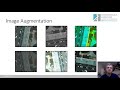
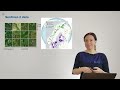
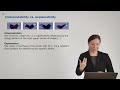
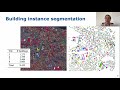
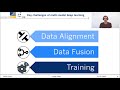
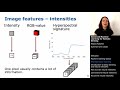
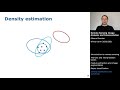
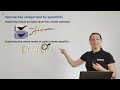
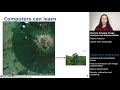
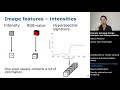
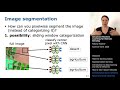
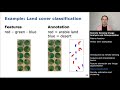