Interpretable latent space and inverse problem in deep generative models
Abstract:
Recent progress in deep generative models such as Generative Adversarial Networks (GANs) has enabled synthesizing photo-realistic images, such as faces and scenes. However, it remains much less explored on what has been learned in the deep generative representation and why diverse realistic images can be synthesized. In this talk, I will present our recent series work from GenForce (https://genforce.github.io/) on interpreting and utilizing latent space of the GANs. Identifying these semantics not only allows us to better understand the inner working of the deep generative models but also facilitates versatile image editings. I will also briefly talk about the inverse problem (how to invert a given image into the latent code) and the fairness of the generative model.
Speaker: Bolei Zhou, The Chinese University of Hong Kong
This seminar was a part of the Spring 2021 ML@GT Seminar Series: http://ml.gatech.edu/seminars
About ML@GT:
The Machine Learning Center was founded in 2016 as an interdisciplinary research center (IRC) at the Georgia Institute of Technology. Since then, we have grown to include over 190 affiliated faculty members and 60 Ph.D. students, all publishing at world-renowned conferences. The center aims to research and develop innovative and sustainable technologies using machine learning and artificial intelligence (AI) that serve our community in socially and ethically responsible ways.
Видео Interpretable latent space and inverse problem in deep generative models канала Machine Learning Center at Georgia Tech
Recent progress in deep generative models such as Generative Adversarial Networks (GANs) has enabled synthesizing photo-realistic images, such as faces and scenes. However, it remains much less explored on what has been learned in the deep generative representation and why diverse realistic images can be synthesized. In this talk, I will present our recent series work from GenForce (https://genforce.github.io/) on interpreting and utilizing latent space of the GANs. Identifying these semantics not only allows us to better understand the inner working of the deep generative models but also facilitates versatile image editings. I will also briefly talk about the inverse problem (how to invert a given image into the latent code) and the fairness of the generative model.
Speaker: Bolei Zhou, The Chinese University of Hong Kong
This seminar was a part of the Spring 2021 ML@GT Seminar Series: http://ml.gatech.edu/seminars
About ML@GT:
The Machine Learning Center was founded in 2016 as an interdisciplinary research center (IRC) at the Georgia Institute of Technology. Since then, we have grown to include over 190 affiliated faculty members and 60 Ph.D. students, all publishing at world-renowned conferences. The center aims to research and develop innovative and sustainable technologies using machine learning and artificial intelligence (AI) that serve our community in socially and ethically responsible ways.
Видео Interpretable latent space and inverse problem in deep generative models канала Machine Learning Center at Georgia Tech
Показать
Комментарии отсутствуют
Информация о видео
11 февраля 2021 г. 0:05:44
00:52:12
Другие видео канала
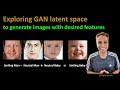


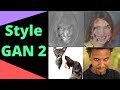
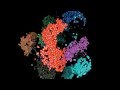
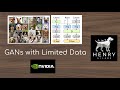
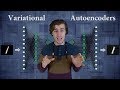

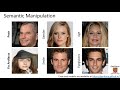
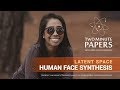
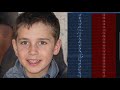
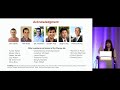
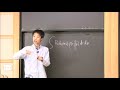
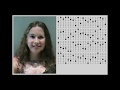
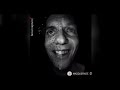
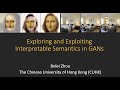

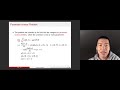
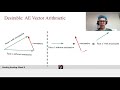
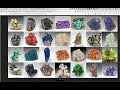