Forough Arabshahi: Neuro-Symbolic Learning Algorithms for Automated Reasoning
Humans possess impressive problem solving and reasoning capabilities, be it mathematical, logical or commonsense reasoning. Computer scientists have long had the dream of building machines with similar reasoning and problem solving abilities as humans. Currently, there are three main challenges in realizing this dream. First, the designed system should be able to extrapolate and reason in scenarios that are much harder than what it has seen before. Second, the system’s decisions/actions should be interpretable, so that humans can easily verify if the decisions are due to reasoning skills or artifacts/sparsity in data. Finally, even if the decisions are easily interpretable, the system should include some way for the user to efficiently teach the correct reasoning when it makes an incorrect decision.
In this talk, I will discuss how we can address these challenges using instructable neuro-symbolic reasoning systems. Neuro-symbolic systems bridge the gap between two major directions in artificial intelligence research: symbolic systems and neural networks. We will see how these hybrid models exploit the interpretability of symbolic systems to obtain explainability. Moreover, combined with our developed neural networks, they extrapolate to harder reasoning problems. Finally, these systems can be directly instructed by humans in natural language, resulting in sample-efficient learning in data-sparse scenarios.
Видео Forough Arabshahi: Neuro-Symbolic Learning Algorithms for Automated Reasoning канала Allen Institute for AI
In this talk, I will discuss how we can address these challenges using instructable neuro-symbolic reasoning systems. Neuro-symbolic systems bridge the gap between two major directions in artificial intelligence research: symbolic systems and neural networks. We will see how these hybrid models exploit the interpretability of symbolic systems to obtain explainability. Moreover, combined with our developed neural networks, they extrapolate to harder reasoning problems. Finally, these systems can be directly instructed by humans in natural language, resulting in sample-efficient learning in data-sparse scenarios.
Видео Forough Arabshahi: Neuro-Symbolic Learning Algorithms for Automated Reasoning канала Allen Institute for AI
Показать
Комментарии отсутствуют
Информация о видео
Другие видео канала
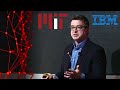
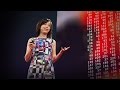
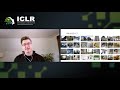
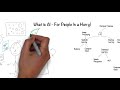
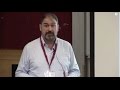
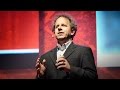
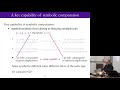


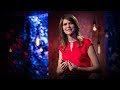
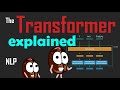
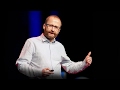
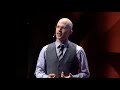
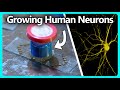
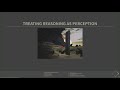
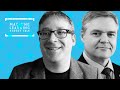
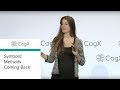
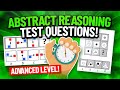
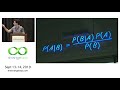
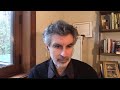