Tom Goldstein: "What do neural loss surfaces look like?"
New Deep Learning Techniques 2018
"What do neural loss surfaces look like?"
Tom Goldstein, University of Maryland
Abstract: Neural network training relies on our ability to find “good” minimizers of highly non-convex loss functions. It is well known that certain network architecture designs (e.g., skip connections) produce loss functions that train easier, and well-chosen training parameters (batch size, learning rate, optimizer) produce minimizers that generalize better. However, the reasons for these differences, and their effects on the underlying loss landscape, are not well understood. In this paper, we explore the structure of neural loss functions, and the effect of loss landscapes on generalization, using a range of visualization methods. First, we introduce a simple “filter normalization” method that helps us visualize loss function curvature, and make meaningful side-by-side comparisons between loss functions. Using this method, we explore how network architecture affects the loss landscape, and how training parameters affect the shape of minimizers.
Institute for Pure and Applied Mathematics, UCLA
February 8, 2018
For more information: http://www.ipam.ucla.edu/programs/workshops/new-deep-learning-techniques/?tab=overview
Видео Tom Goldstein: "What do neural loss surfaces look like?" канала Institute for Pure & Applied Mathematics (IPAM)
"What do neural loss surfaces look like?"
Tom Goldstein, University of Maryland
Abstract: Neural network training relies on our ability to find “good” minimizers of highly non-convex loss functions. It is well known that certain network architecture designs (e.g., skip connections) produce loss functions that train easier, and well-chosen training parameters (batch size, learning rate, optimizer) produce minimizers that generalize better. However, the reasons for these differences, and their effects on the underlying loss landscape, are not well understood. In this paper, we explore the structure of neural loss functions, and the effect of loss landscapes on generalization, using a range of visualization methods. First, we introduce a simple “filter normalization” method that helps us visualize loss function curvature, and make meaningful side-by-side comparisons between loss functions. Using this method, we explore how network architecture affects the loss landscape, and how training parameters affect the shape of minimizers.
Institute for Pure and Applied Mathematics, UCLA
February 8, 2018
For more information: http://www.ipam.ucla.edu/programs/workshops/new-deep-learning-techniques/?tab=overview
Видео Tom Goldstein: "What do neural loss surfaces look like?" канала Institute for Pure & Applied Mathematics (IPAM)
Показать
Комментарии отсутствуют
Информация о видео
17 февраля 2018 г. 4:06:04
00:50:26
Другие видео канала
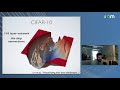
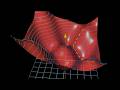
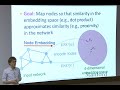
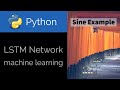
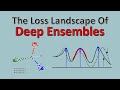

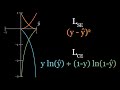
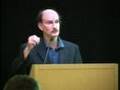
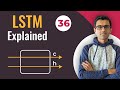
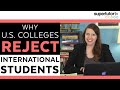
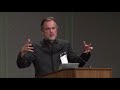
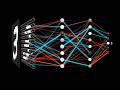
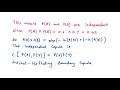
![Lecture 43 — Introduction to Information Extraction — [ NLP || Christopher Manning || Stanford ]](https://i.ytimg.com/vi/Tj3Dkiw-iZg/default.jpg)
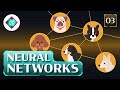
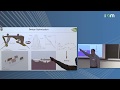
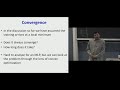
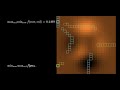
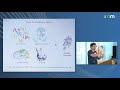
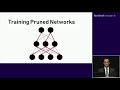