NeuralHash is BROKEN - How to evade Apple's detection & craft hash collisions (w/ Open Source Code)
#apple #icloud #neuralhash
Send your Apple fanboy friends to prison with this one simple trick ;) We break Apple's NeuralHash algorithm used to detect CSAM for iCloud photos. I show how it's possible to craft arbitrary hash collisions from any source / target image pair using an adversarial example attack. This can be used for many purposes, such as evading detection, or forging false positives, triggering manual reviews.
OUTLINE:
0:00 - Intro
1:30 - Forced Hash Collisions via Adversarial Attacks
2:30 - My Successful Attack
5:40 - Results
7:15 - Discussion
DISCLAIMER: This is for demonstration and educational purposes only. This is not an endorsement of illegal activity or circumvention of law.
Code: https://github.com/yk/neural_hash_collision
Extract Model: https://github.com/AsuharietYgvar/AppleNeuralHash2ONNX
My Video on NeuralHash: https://youtu.be/z15JLtAuwVI
ADDENDUM:
The application of framing people is a bit more intricate than I point out here. Apple has commented that there would be a second perceptual hashing scheme server-side, i.e. the model would not be released, which makes forging false positives harder. Nevertheless, evading the system remains fairly trivial.
Links:
TabNine Code Completion (Referral): http://bit.ly/tabnine-yannick
YouTube: https://www.youtube.com/c/yannickilcher
Twitter: https://twitter.com/ykilcher
Discord: https://discord.gg/4H8xxDF
BitChute: https://www.bitchute.com/channel/yannic-kilcher
Minds: https://www.minds.com/ykilcher
Parler: https://parler.com/profile/YannicKilcher
LinkedIn: https://www.linkedin.com/in/yannic-kilcher-488534136/
BiliBili: https://space.bilibili.com/1824646584
If you want to support me, the best thing to do is to share out the content :)
If you want to support me financially (completely optional and voluntary, but a lot of people have asked for this):
SubscribeStar: https://www.subscribestar.com/yannickilcher
Patreon: https://www.patreon.com/yannickilcher
Bitcoin (BTC): bc1q49lsw3q325tr58ygf8sudx2dqfguclvngvy2cq
Ethereum (ETH): 0x7ad3513E3B8f66799f507Aa7874b1B0eBC7F85e2
Litecoin (LTC): LQW2TRyKYetVC8WjFkhpPhtpbDM4Vw7r9m
Monero (XMR): 4ACL8AGrEo5hAir8A9CeVrW8pEauWvnp1WnSDZxW7tziCDLhZAGsgzhRQABDnFy8yuM9fWJDviJPHKRjV4FWt19CJZN9D4n
Видео NeuralHash is BROKEN - How to evade Apple's detection & craft hash collisions (w/ Open Source Code) канала Yannic Kilcher
Send your Apple fanboy friends to prison with this one simple trick ;) We break Apple's NeuralHash algorithm used to detect CSAM for iCloud photos. I show how it's possible to craft arbitrary hash collisions from any source / target image pair using an adversarial example attack. This can be used for many purposes, such as evading detection, or forging false positives, triggering manual reviews.
OUTLINE:
0:00 - Intro
1:30 - Forced Hash Collisions via Adversarial Attacks
2:30 - My Successful Attack
5:40 - Results
7:15 - Discussion
DISCLAIMER: This is for demonstration and educational purposes only. This is not an endorsement of illegal activity or circumvention of law.
Code: https://github.com/yk/neural_hash_collision
Extract Model: https://github.com/AsuharietYgvar/AppleNeuralHash2ONNX
My Video on NeuralHash: https://youtu.be/z15JLtAuwVI
ADDENDUM:
The application of framing people is a bit more intricate than I point out here. Apple has commented that there would be a second perceptual hashing scheme server-side, i.e. the model would not be released, which makes forging false positives harder. Nevertheless, evading the system remains fairly trivial.
Links:
TabNine Code Completion (Referral): http://bit.ly/tabnine-yannick
YouTube: https://www.youtube.com/c/yannickilcher
Twitter: https://twitter.com/ykilcher
Discord: https://discord.gg/4H8xxDF
BitChute: https://www.bitchute.com/channel/yannic-kilcher
Minds: https://www.minds.com/ykilcher
Parler: https://parler.com/profile/YannicKilcher
LinkedIn: https://www.linkedin.com/in/yannic-kilcher-488534136/
BiliBili: https://space.bilibili.com/1824646584
If you want to support me, the best thing to do is to share out the content :)
If you want to support me financially (completely optional and voluntary, but a lot of people have asked for this):
SubscribeStar: https://www.subscribestar.com/yannickilcher
Patreon: https://www.patreon.com/yannickilcher
Bitcoin (BTC): bc1q49lsw3q325tr58ygf8sudx2dqfguclvngvy2cq
Ethereum (ETH): 0x7ad3513E3B8f66799f507Aa7874b1B0eBC7F85e2
Litecoin (LTC): LQW2TRyKYetVC8WjFkhpPhtpbDM4Vw7r9m
Monero (XMR): 4ACL8AGrEo5hAir8A9CeVrW8pEauWvnp1WnSDZxW7tziCDLhZAGsgzhRQABDnFy8yuM9fWJDviJPHKRjV4FWt19CJZN9D4n
Видео NeuralHash is BROKEN - How to evade Apple's detection & craft hash collisions (w/ Open Source Code) канала Yannic Kilcher
Показать
Комментарии отсутствуют
Информация о видео
Другие видео канала
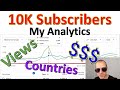
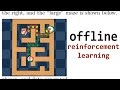
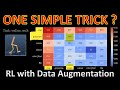
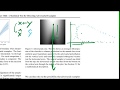
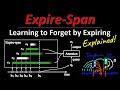
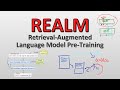
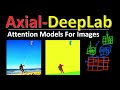
![[Classic] Playing Atari with Deep Reinforcement Learning (Paper Explained)](https://i.ytimg.com/vi/rFwQDDbYTm4/default.jpg)
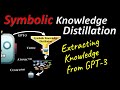
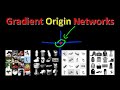
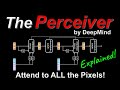
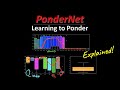
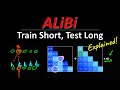
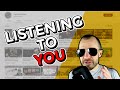
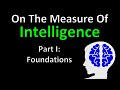
![[ML News] Uber: Deep Learning for ETA | MuZero Video Compression | Block-NeRF | EfficientNet-X](https://i.ytimg.com/vi/fEKZC9mta8w/default.jpg)
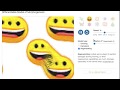
![[ML News] DeepMind's Flamingo Image-Text model | Locked-Image Tuning | Jurassic X & MRKL](https://i.ytimg.com/vi/smUHQndcmOY/default.jpg)
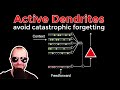
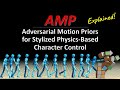
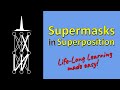