Optimizing SQL Queries: How to Improve Your select count for PostgreSQL Arrays
Discover effective strategies to optimize your SQL queries in PostgreSQL, focusing on `select count` for large tables with array comparisons.
---
This video is based on the question https://stackoverflow.com/q/74884863/ asked by the user 'Siwei' ( https://stackoverflow.com/u/445908/ ) and on the answer https://stackoverflow.com/a/74887967/ provided by the user 'jjanes' ( https://stackoverflow.com/u/1721239/ ) at 'Stack Overflow' website. Thanks to these great users and Stackexchange community for their contributions.
Visit these links for original content and any more details, such as alternate solutions, latest updates/developments on topic, comments, revision history etc. For example, the original title of the Question was: How to optimize this "select count" SQL? (postgres array comparision)
Also, Content (except music) licensed under CC BY-SA https://meta.stackexchange.com/help/licensing
The original Question post is licensed under the 'CC BY-SA 4.0' ( https://creativecommons.org/licenses/by-sa/4.0/ ) license, and the original Answer post is licensed under the 'CC BY-SA 4.0' ( https://creativecommons.org/licenses/by-sa/4.0/ ) license.
If anything seems off to you, please feel free to write me at vlogize [AT] gmail [DOT] com.
---
Optimizing SQL Queries: How to Improve Your select count for PostgreSQL Arrays
When dealing with large datasets, every millisecond counts. If you've ever executed a select count SQL query on a massive table and found yourself waiting an unacceptable amount of time, you're not alone. In this guide, we'll dive into a specific scenario: counting records in a PostgreSQL table where a column is an array data type. We'll look at how to optimize this type of query and provide you with practical solutions for better performance.
The Problem Statement
Imagine you have a table named my_table with 10 million records. The relevant structure of the table includes an id, content, and contained_special_ids which is an array type column. Here’s a simplified version of such a structure:
[[See Video to Reveal this Text or Code Snippet]]
The challenge is clear: you need to count how many records have the value 3 within the contained_special_ids column. While the SQL query below may work for smaller datasets:
[[See Video to Reveal this Text or Code Snippet]]
Running this on a larger dataset quickly becomes problematic, taking over 30 seconds for execution, leading to performance concerns for applications relying on fast responses.
Analyzing the Current Performance
To understand why this query runs slowly, we can utilize the EXPLAIN command in PostgreSQL, which reveals the performance metrics of the query execution. In a scenario where the query execution takes over 44 seconds, we can identify targeting areas for optimization. For example, parallel bitmap heap scans show that there are a significant number of rows being removed by index rechecks, indicating inefficiencies in how Postgres accesses the data.
Solutions for Optimization
1. Increase Work Memory
One of the simplest methods to reduce query processing time is to increase the work_mem setting in PostgreSQL. By allocating more memory for internal sorting and hash tables, you can help to reduce the reliance on disk access. This adjustment can decrease the number of lossy blocks during index scans and improve query performance.
2. Regularly Vacuum Your Database
Vacuuming your database is crucial. When you run a VACUUM, PostgreSQL cleans up dead tuples, ensuring that the database statistics are accurate, which helps improve query planning. A well-vacuumed database is essential for leveraging index-only bitmap scans effectively, offering optimal query performance.
3. Update PostgreSQL Version
Ensure that you’re using a recent version of PostgreSQL. Newer versions continually come with performance improvements and features that can enhance how data is handled, especially with large datasets. Features like indexed scans and parallel queries can significantly reduce execution times.
4. Optimize IO Concurrency
Increasing effective_io_concurrency can help optimize query performance as it allows more concurrent I/O operations. This setting is especially beneficial during large data scans, helping to keep your queries running efficiently.
5. Use Descriptive Plans and Track I/O Timing
When sharing your SQL execution plans for further analysis, it's more helpful to post them as text rather than images. Also, turning on track_io_timing can provide crucial insight into how much time your queries are spending on disk I/O, furthering your understanding of where the bottlenecks lie.
Conclusion
Optimizing select count queries, especially when working with array data types in PostgreSQL, can involve several strategic adjustments ranging from configuration changes to maintenance tasks. By implementing these solutions, you can significantly reduce the execution time of your queries, making your applications more responsive and efficient.
Видео Optimizing SQL Queries: How to Improve Your select count for PostgreSQL Arrays канала vlogize
---
This video is based on the question https://stackoverflow.com/q/74884863/ asked by the user 'Siwei' ( https://stackoverflow.com/u/445908/ ) and on the answer https://stackoverflow.com/a/74887967/ provided by the user 'jjanes' ( https://stackoverflow.com/u/1721239/ ) at 'Stack Overflow' website. Thanks to these great users and Stackexchange community for their contributions.
Visit these links for original content and any more details, such as alternate solutions, latest updates/developments on topic, comments, revision history etc. For example, the original title of the Question was: How to optimize this "select count" SQL? (postgres array comparision)
Also, Content (except music) licensed under CC BY-SA https://meta.stackexchange.com/help/licensing
The original Question post is licensed under the 'CC BY-SA 4.0' ( https://creativecommons.org/licenses/by-sa/4.0/ ) license, and the original Answer post is licensed under the 'CC BY-SA 4.0' ( https://creativecommons.org/licenses/by-sa/4.0/ ) license.
If anything seems off to you, please feel free to write me at vlogize [AT] gmail [DOT] com.
---
Optimizing SQL Queries: How to Improve Your select count for PostgreSQL Arrays
When dealing with large datasets, every millisecond counts. If you've ever executed a select count SQL query on a massive table and found yourself waiting an unacceptable amount of time, you're not alone. In this guide, we'll dive into a specific scenario: counting records in a PostgreSQL table where a column is an array data type. We'll look at how to optimize this type of query and provide you with practical solutions for better performance.
The Problem Statement
Imagine you have a table named my_table with 10 million records. The relevant structure of the table includes an id, content, and contained_special_ids which is an array type column. Here’s a simplified version of such a structure:
[[See Video to Reveal this Text or Code Snippet]]
The challenge is clear: you need to count how many records have the value 3 within the contained_special_ids column. While the SQL query below may work for smaller datasets:
[[See Video to Reveal this Text or Code Snippet]]
Running this on a larger dataset quickly becomes problematic, taking over 30 seconds for execution, leading to performance concerns for applications relying on fast responses.
Analyzing the Current Performance
To understand why this query runs slowly, we can utilize the EXPLAIN command in PostgreSQL, which reveals the performance metrics of the query execution. In a scenario where the query execution takes over 44 seconds, we can identify targeting areas for optimization. For example, parallel bitmap heap scans show that there are a significant number of rows being removed by index rechecks, indicating inefficiencies in how Postgres accesses the data.
Solutions for Optimization
1. Increase Work Memory
One of the simplest methods to reduce query processing time is to increase the work_mem setting in PostgreSQL. By allocating more memory for internal sorting and hash tables, you can help to reduce the reliance on disk access. This adjustment can decrease the number of lossy blocks during index scans and improve query performance.
2. Regularly Vacuum Your Database
Vacuuming your database is crucial. When you run a VACUUM, PostgreSQL cleans up dead tuples, ensuring that the database statistics are accurate, which helps improve query planning. A well-vacuumed database is essential for leveraging index-only bitmap scans effectively, offering optimal query performance.
3. Update PostgreSQL Version
Ensure that you’re using a recent version of PostgreSQL. Newer versions continually come with performance improvements and features that can enhance how data is handled, especially with large datasets. Features like indexed scans and parallel queries can significantly reduce execution times.
4. Optimize IO Concurrency
Increasing effective_io_concurrency can help optimize query performance as it allows more concurrent I/O operations. This setting is especially beneficial during large data scans, helping to keep your queries running efficiently.
5. Use Descriptive Plans and Track I/O Timing
When sharing your SQL execution plans for further analysis, it's more helpful to post them as text rather than images. Also, turning on track_io_timing can provide crucial insight into how much time your queries are spending on disk I/O, furthering your understanding of where the bottlenecks lie.
Conclusion
Optimizing select count queries, especially when working with array data types in PostgreSQL, can involve several strategic adjustments ranging from configuration changes to maintenance tasks. By implementing these solutions, you can significantly reduce the execution time of your queries, making your applications more responsive and efficient.
Видео Optimizing SQL Queries: How to Improve Your select count for PostgreSQL Arrays канала vlogize
Комментарии отсутствуют
Информация о видео
1 апреля 2025 г. 10:14:11
00:01:43
Другие видео канала

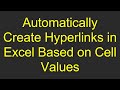
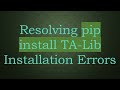

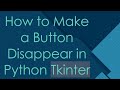
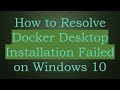


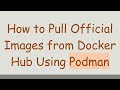
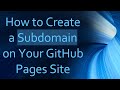
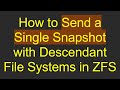

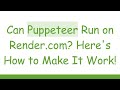




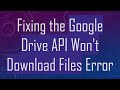
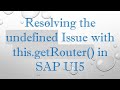

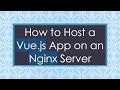