Max Welling: Intelligence per Kilowatthour (ICML 2018 invited talk)
Abstract: In the 19th century the world was revolutionized because we could transform energy into useful work. The 21st century is revolutionized due to our ability to transform information (or data) into useful tools. Driven by Moore's law and the exponential growth of data, artificial intelligence is permeating every aspect of our lives. But intelligence is not for free, it costs energy, and therefore money. Evolution has faced this problem for millions of years and made brains about a 100x more energy efficient than modern hardware (or, as in the case of the sea-squirt, decided that it should eat its brain once is was no longer necessary). I will argue that energy will soon be one of the determining factors in AI. Either companies will find it too expensive to run energy hungry ML tools (such as deep learning) to power their AI engines, or the heat dissipation in edge devices will be too high to be safe. The next battleground in AI might well be a race for the most energy efficient combination of hardware and algorithms.
In this talk I will discuss some ideas that could address this problem. The technical hammer that I will exploit is the perfect reflection of the energy versus information balancing act we must address: the free energy, which is the expected energy minus the entropy of a system. Using the free energy we develop a Bayesian interpretation of deep learning which, with the appropriate sparsity inducing priors, can be used to prune both neurons and quantize parameters to low precision. The second hammer I will exploit is sigma-delta modulation (also known as herding) to introduce spiking into deep learning in an attempt to avoid computation in the absence of changes.
Видео Max Welling: Intelligence per Kilowatthour (ICML 2018 invited talk) канала Steven Van Vaerenbergh
In this talk I will discuss some ideas that could address this problem. The technical hammer that I will exploit is the perfect reflection of the energy versus information balancing act we must address: the free energy, which is the expected energy minus the entropy of a system. Using the free energy we develop a Bayesian interpretation of deep learning which, with the appropriate sparsity inducing priors, can be used to prune both neurons and quantize parameters to low precision. The second hammer I will exploit is sigma-delta modulation (also known as herding) to introduce spiking into deep learning in an attempt to avoid computation in the absence of changes.
Видео Max Welling: Intelligence per Kilowatthour (ICML 2018 invited talk) канала Steven Van Vaerenbergh
Показать
Комментарии отсутствуют
Информация о видео
Другие видео канала
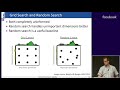
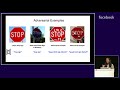
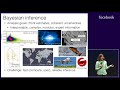
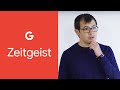
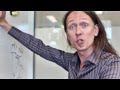
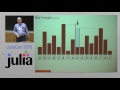

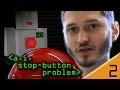

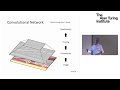
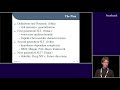
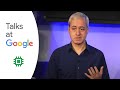
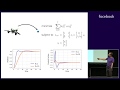
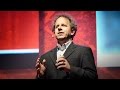

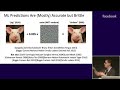
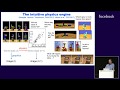
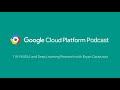
![[DeepBayes2018]: Day 3, Invited talk 1. Advanced methods of variational inference](https://i.ytimg.com/vi/mCBnid-1slI/default.jpg)
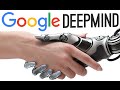