Scaling Transformer to 1M tokens and beyond with RMT (Paper Explained)
#ai #transformer #gpt4
This paper promises to scale transformers to 1 million tokens and beyond. We take a look at the technique behind it: The Recurrent Memory Transformer, and what its strenghts and weaknesses are.
OUTLINE:
0:00 - Intro
2:15 - Transformers on long sequences
4:30 - Tasks considered
8:00 - Recurrent Memory Transformer
19:40 - Experiments on scaling and attention maps
24:00 - Conclusion
Paper: https://arxiv.org/abs/2304.11062
Abstract:
This technical report presents the application of a recurrent memory to extend the context length of BERT, one of the most effective Transformer-based models in natural language processing. By leveraging the Recurrent Memory Transformer architecture, we have successfully increased the model's effective context length to an unprecedented two million tokens, while maintaining high memory retrieval accuracy. Our method allows for the storage and processing of both local and global information and enables information flow between segments of the input sequence through the use of recurrence. Our experiments demonstrate the effectiveness of our approach, which holds significant potential to enhance long-term dependency handling in natural language understanding and generation tasks as well as enable large-scale context processing for memory-intensive applications.
Authors: Aydar Bulatov, Yuri Kuratov, Mikhail S. Burtsev
Links:
Homepage: https://ykilcher.com
Merch: https://ykilcher.com/merch
YouTube: https://www.youtube.com/c/yannickilcher
Twitter: https://twitter.com/ykilcher
Discord: https://ykilcher.com/discord
LinkedIn: https://www.linkedin.com/in/ykilcher
If you want to support me, the best thing to do is to share out the content :)
If you want to support me financially (completely optional and voluntary, but a lot of people have asked for this):
SubscribeStar: https://www.subscribestar.com/yannickilcher
Patreon: https://www.patreon.com/yannickilcher
Bitcoin (BTC): bc1q49lsw3q325tr58ygf8sudx2dqfguclvngvy2cq
Ethereum (ETH): 0x7ad3513E3B8f66799f507Aa7874b1B0eBC7F85e2
Litecoin (LTC): LQW2TRyKYetVC8WjFkhpPhtpbDM4Vw7r9m
Monero (XMR): 4ACL8AGrEo5hAir8A9CeVrW8pEauWvnp1WnSDZxW7tziCDLhZAGsgzhRQABDnFy8yuM9fWJDviJPHKRjV4FWt19CJZN9D4n
Видео Scaling Transformer to 1M tokens and beyond with RMT (Paper Explained) канала Yannic Kilcher
This paper promises to scale transformers to 1 million tokens and beyond. We take a look at the technique behind it: The Recurrent Memory Transformer, and what its strenghts and weaknesses are.
OUTLINE:
0:00 - Intro
2:15 - Transformers on long sequences
4:30 - Tasks considered
8:00 - Recurrent Memory Transformer
19:40 - Experiments on scaling and attention maps
24:00 - Conclusion
Paper: https://arxiv.org/abs/2304.11062
Abstract:
This technical report presents the application of a recurrent memory to extend the context length of BERT, one of the most effective Transformer-based models in natural language processing. By leveraging the Recurrent Memory Transformer architecture, we have successfully increased the model's effective context length to an unprecedented two million tokens, while maintaining high memory retrieval accuracy. Our method allows for the storage and processing of both local and global information and enables information flow between segments of the input sequence through the use of recurrence. Our experiments demonstrate the effectiveness of our approach, which holds significant potential to enhance long-term dependency handling in natural language understanding and generation tasks as well as enable large-scale context processing for memory-intensive applications.
Authors: Aydar Bulatov, Yuri Kuratov, Mikhail S. Burtsev
Links:
Homepage: https://ykilcher.com
Merch: https://ykilcher.com/merch
YouTube: https://www.youtube.com/c/yannickilcher
Twitter: https://twitter.com/ykilcher
Discord: https://ykilcher.com/discord
LinkedIn: https://www.linkedin.com/in/ykilcher
If you want to support me, the best thing to do is to share out the content :)
If you want to support me financially (completely optional and voluntary, but a lot of people have asked for this):
SubscribeStar: https://www.subscribestar.com/yannickilcher
Patreon: https://www.patreon.com/yannickilcher
Bitcoin (BTC): bc1q49lsw3q325tr58ygf8sudx2dqfguclvngvy2cq
Ethereum (ETH): 0x7ad3513E3B8f66799f507Aa7874b1B0eBC7F85e2
Litecoin (LTC): LQW2TRyKYetVC8WjFkhpPhtpbDM4Vw7r9m
Monero (XMR): 4ACL8AGrEo5hAir8A9CeVrW8pEauWvnp1WnSDZxW7tziCDLhZAGsgzhRQABDnFy8yuM9fWJDviJPHKRjV4FWt19CJZN9D4n
Видео Scaling Transformer to 1M tokens and beyond with RMT (Paper Explained) канала Yannic Kilcher
Показать
Комментарии отсутствуют
Информация о видео
Другие видео канала
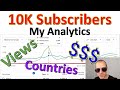
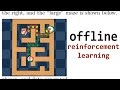
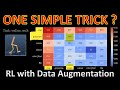
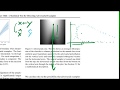
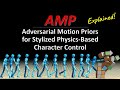
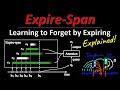
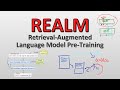
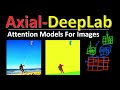
![[Classic] Playing Atari with Deep Reinforcement Learning (Paper Explained)](https://i.ytimg.com/vi/rFwQDDbYTm4/default.jpg)
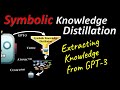
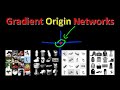
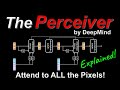
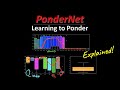
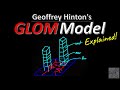
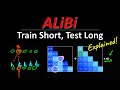
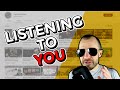
![[ML News] Uber: Deep Learning for ETA | MuZero Video Compression | Block-NeRF | EfficientNet-X](https://i.ytimg.com/vi/fEKZC9mta8w/default.jpg)
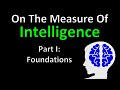
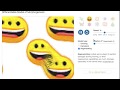
![[ML News] DeepMind's Flamingo Image-Text model | Locked-Image Tuning | Jurassic X & MRKL](https://i.ytimg.com/vi/smUHQndcmOY/default.jpg)
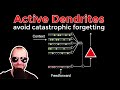