Real-Time Panoptic Segmentation From Dense Detections
Authors: Rui Hou, Jie Li, Arjun Bhargava, Allan Raventos, Vitor Guizilini, Chao Fang, Jerome Lynch, Adrien Gaidon Description: Panoptic segmentation is a complex full scene parsing task requiring simultaneous instance and semantic segmentation at high resolution. Current state-of-the-art approaches cannot run in real-time, and simplifying these architectures to improve efficiency severely degrades their accuracy. In this paper, we propose a new single-shot panoptic segmentation network that leverages dense detections and a global self-attention mechanism to operate in real-time with performance approaching the state of the art. We introduce a novel parameter-free mask construction method that substantially reduces computational complexity by efficiently reusing information from the object detection and semantic segmentation sub-tasks. The resulting network has a simple data flow that requires no feature map re-sampling, enabling significant hardware acceleration. Our experiments on the Cityscapes and COCO benchmarks show that our network works at 30 FPS on 1024x2048 resolution, trading a 3% relative performance degradation from the current state of the art for up to 440% faster inference.
Видео Real-Time Panoptic Segmentation From Dense Detections канала ComputerVisionFoundation Videos
Видео Real-Time Panoptic Segmentation From Dense Detections канала ComputerVisionFoundation Videos
Показать
Комментарии отсутствуют
Информация о видео
17 июля 2020 г. 13:20:08
00:04:49
Другие видео канала

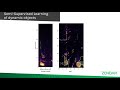
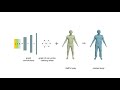
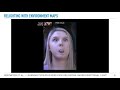
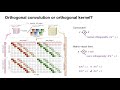
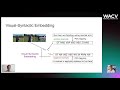
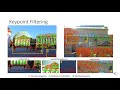
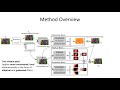
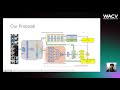
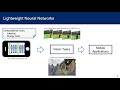
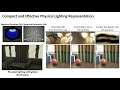
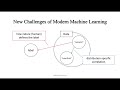
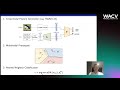
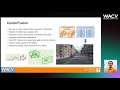


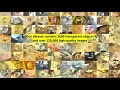
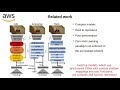
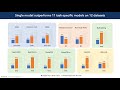
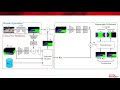
