EI Seminar - Michael Carbin - The Lottery Ticket Hypothesis
Title: The Lottery Ticket Hypothesis: Finding Sparse, Trainable Neural Networks
Abstract: Neural network pruning techniques can reduce the parameter counts of trained networks by over 90%, decreasing storage requirements and improving computational performance of inference without compromising accuracy. However, contemporary experience is that the sparse architectures produced by pruning are difficult to train from the start and, instead, training must first begin with large, overparameterized networks.
In this talk, I’ll present our work on The Lottery Ticket Hypothesis, showing that at a standard pruning technique, iterative magnitude pruning, naturally uncovers subnetworks that are capable of training effectively from early in training. These subnetworks hold out the promise of more efficient machine learning methods, including inference, fine-tuning of pre-trained networks, and sparse training.
Bio: Michael Carbin is an assistant professor in MIT’s Department of Electrical Engineering and Computer Science and a principal investigator at the Computer Science and Artificial Intelligence Laboratory, where he leads the Programming Systems Group. His group investigates the semantics, design, and implementation of systems that operate in the presence of uncertainty in their environment (perception), implementation (neural networks or approximate transformations), or execution (unreliable hardware). Carbin has received a Sloan Research Fellowship, a Facebook Research Award, a Google Faculty Research Award and an NSF Career Award. He earned a BS in computer science at Stanford University and an MS and PhD in electrical engineering and computer science from MIT.
Видео EI Seminar - Michael Carbin - The Lottery Ticket Hypothesis канала MIT Embodied Intelligence
Abstract: Neural network pruning techniques can reduce the parameter counts of trained networks by over 90%, decreasing storage requirements and improving computational performance of inference without compromising accuracy. However, contemporary experience is that the sparse architectures produced by pruning are difficult to train from the start and, instead, training must first begin with large, overparameterized networks.
In this talk, I’ll present our work on The Lottery Ticket Hypothesis, showing that at a standard pruning technique, iterative magnitude pruning, naturally uncovers subnetworks that are capable of training effectively from early in training. These subnetworks hold out the promise of more efficient machine learning methods, including inference, fine-tuning of pre-trained networks, and sparse training.
Bio: Michael Carbin is an assistant professor in MIT’s Department of Electrical Engineering and Computer Science and a principal investigator at the Computer Science and Artificial Intelligence Laboratory, where he leads the Programming Systems Group. His group investigates the semantics, design, and implementation of systems that operate in the presence of uncertainty in their environment (perception), implementation (neural networks or approximate transformations), or execution (unreliable hardware). Carbin has received a Sloan Research Fellowship, a Facebook Research Award, a Google Faculty Research Award and an NSF Career Award. He earned a BS in computer science at Stanford University and an MS and PhD in electrical engineering and computer science from MIT.
Видео EI Seminar - Michael Carbin - The Lottery Ticket Hypothesis канала MIT Embodied Intelligence
Показать
Комментарии отсутствуют
Информация о видео
Другие видео канала
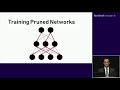
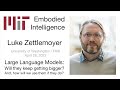
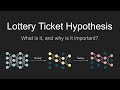
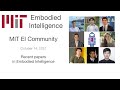
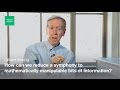
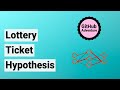
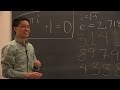
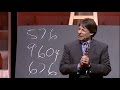
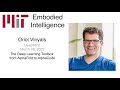
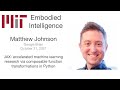
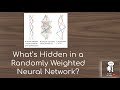
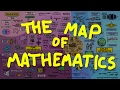
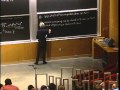

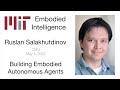
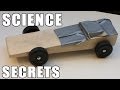
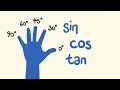

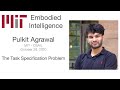
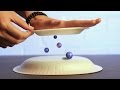