Descriptive vs Predictive vs Prescriptive Analytics
Subscribe to RichardOnData here: https://www.youtube.com/channel/UCKPyg5gsnt6h0aA8EBw3i6A?sub_confirmation=1
In this video I break down the difference between and provide examples of descriptive, predictive, and prescriptive analytics. These are terms proliferated in the business analytics, statistics, and data science worlds, typically presented in a pipeline with descriptive occurring first, predictive occurring next, and prescriptive occurring last.
These are not a comprehensive list of examples of these types of analytics, but rather intended to illustrate the scope and breadth of these forms of analytics as well as provide some helpful, practical ideas.
DESCRIPTIVE ANALYTICS:
These describe to an end-user what HAS HAPPENED IN THE PAST. This is by far and away the most common form of analytic -- and will play at least a small if not huge role in your day-to-day if you are a data scientist. Descriptive analytics include: summary metrics like means, medians, sums, totals, percents, etc., as well as graphs like bar charts, histograms, box plots, time plots, and pie charts. They also include statistical methods like confidence intervals, hypothesis tests, clustering algorithms, and linear/logistic regression when they are applied with the intention of inference. Note that these analytics are NOT necessarily predictive or prescriptive even if a human's instinct is to infer that such things will happen in the future, or make a business decision based on them.
PREDICTIVE ANALYTICS:
These provide insight into WHAT WILL HAPPEN IN THE FUTURE. As a general rule, forecasting models as well as classification or regression models where the intent is prediction rather than inference, will fall into this category. There are predictive analytic variants for spatial data as well as time-series data (e.g. ARIMA -- Autoregressive Integrated Moving Average models). Lastly, supervised learning models for regression/classification and deep learning algorithms fall in this category as well. Simpler types of predictive analytics can be built with business rules; however these are common examples because they all control for variation in the system.
PRESCRIPTIVE ANALYTICS:
These are the "holy grail" of analytics, designed to tell an end user WHAT THEY SHOULD DO. These generally operate by generating various outcomes from different scenarios, and then selecting the best one by controlling for various factors and uncertainty. Probably the most common form is a mathematical optimization model which optimizes an objective function subject to some outcome, features, and constraints. These are also a variety of simulation models: Monte Carlo simulations which use repeated random sampling to control for features which a lot of variation/uncertainty; and discrete event simulations where a real-life process and all of its actions/items and their interactions can be created and impacts compared. Again, you can make a simpler version using business rules and assumptions if time is short or one is unfamiliar with more complex methods.
Lastly, it may be a bit unrealistic to think of these as a pipeline, because in practice, utilizing them can feel a lot more like a cycle. Predictive analytics can easily lend themselves to new ideas for descriptive analytics, and so on.
#DescriptiveAnalytics #PredictiveAnalytics #PrescriptiveAnalytics
PayPal: richardondata@gmail.com
Patreon: https://www.patreon.com/richardondata
BTC: 3LM5d1vibhp1F7pcxAFX8Ys1DM6XLUoNVL
ETH: 0x3CfC599C4c1040963B644780a0E62d45999bE9D8
LTC: MH8yPjvSmKvpmRRmufofjRB9hnRAFHfx32
Видео Descriptive vs Predictive vs Prescriptive Analytics канала RichardOnData
In this video I break down the difference between and provide examples of descriptive, predictive, and prescriptive analytics. These are terms proliferated in the business analytics, statistics, and data science worlds, typically presented in a pipeline with descriptive occurring first, predictive occurring next, and prescriptive occurring last.
These are not a comprehensive list of examples of these types of analytics, but rather intended to illustrate the scope and breadth of these forms of analytics as well as provide some helpful, practical ideas.
DESCRIPTIVE ANALYTICS:
These describe to an end-user what HAS HAPPENED IN THE PAST. This is by far and away the most common form of analytic -- and will play at least a small if not huge role in your day-to-day if you are a data scientist. Descriptive analytics include: summary metrics like means, medians, sums, totals, percents, etc., as well as graphs like bar charts, histograms, box plots, time plots, and pie charts. They also include statistical methods like confidence intervals, hypothesis tests, clustering algorithms, and linear/logistic regression when they are applied with the intention of inference. Note that these analytics are NOT necessarily predictive or prescriptive even if a human's instinct is to infer that such things will happen in the future, or make a business decision based on them.
PREDICTIVE ANALYTICS:
These provide insight into WHAT WILL HAPPEN IN THE FUTURE. As a general rule, forecasting models as well as classification or regression models where the intent is prediction rather than inference, will fall into this category. There are predictive analytic variants for spatial data as well as time-series data (e.g. ARIMA -- Autoregressive Integrated Moving Average models). Lastly, supervised learning models for regression/classification and deep learning algorithms fall in this category as well. Simpler types of predictive analytics can be built with business rules; however these are common examples because they all control for variation in the system.
PRESCRIPTIVE ANALYTICS:
These are the "holy grail" of analytics, designed to tell an end user WHAT THEY SHOULD DO. These generally operate by generating various outcomes from different scenarios, and then selecting the best one by controlling for various factors and uncertainty. Probably the most common form is a mathematical optimization model which optimizes an objective function subject to some outcome, features, and constraints. These are also a variety of simulation models: Monte Carlo simulations which use repeated random sampling to control for features which a lot of variation/uncertainty; and discrete event simulations where a real-life process and all of its actions/items and their interactions can be created and impacts compared. Again, you can make a simpler version using business rules and assumptions if time is short or one is unfamiliar with more complex methods.
Lastly, it may be a bit unrealistic to think of these as a pipeline, because in practice, utilizing them can feel a lot more like a cycle. Predictive analytics can easily lend themselves to new ideas for descriptive analytics, and so on.
#DescriptiveAnalytics #PredictiveAnalytics #PrescriptiveAnalytics
PayPal: richardondata@gmail.com
Patreon: https://www.patreon.com/richardondata
BTC: 3LM5d1vibhp1F7pcxAFX8Ys1DM6XLUoNVL
ETH: 0x3CfC599C4c1040963B644780a0E62d45999bE9D8
LTC: MH8yPjvSmKvpmRRmufofjRB9hnRAFHfx32
Видео Descriptive vs Predictive vs Prescriptive Analytics канала RichardOnData
Показать
Комментарии отсутствуют
Информация о видео
Другие видео канала
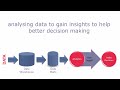


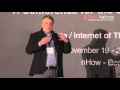

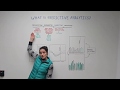

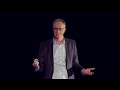
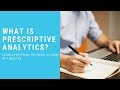
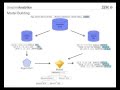
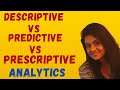
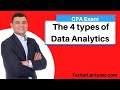
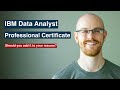
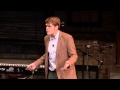
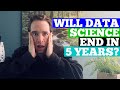
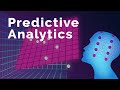

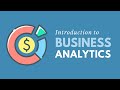

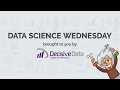