RoBERTa: A Robustly Optimized BERT Pretraining Approach
This paper shows that the original BERT model, if trained correctly, can outperform all of the improvements that have been proposed lately, raising questions about the necessity and reasoning behind these.
Abstract:
Language model pretraining has led to significant performance gains but careful comparison between different approaches is challenging. Training is computationally expensive, often done on private datasets of different sizes, and, as we will show, hyperparameter choices have significant impact on the final results. We present a replication study of BERT pretraining (Devlin et al., 2019) that carefully measures the impact of many key hyperparameters and training data size. We find that BERT was significantly undertrained, and can match or exceed the performance of every model published after it. Our best model achieves state-of-the-art results on GLUE, RACE and SQuAD. These results highlight the importance of previously overlooked design choices, and raise questions about the source of recently reported improvements. We release our models and code.
Authors: Yinhan Liu, Myle Ott, Naman Goyal, Jingfei Du, Mandar Joshi, Danqi Chen, Omer Levy, Mike Lewis, Luke Zettlemoyer, Veselin Stoyanov
https://arxiv.org/abs/1907.11692
YouTube: https://www.youtube.com/c/yannickilcher
Twitter: https://twitter.com/ykilcher
Minds: https://www.minds.com/ykilcher
BitChute: https://www.bitchute.com/channel/10a5ui845DOJ/
Видео RoBERTa: A Robustly Optimized BERT Pretraining Approach канала Yannic Kilcher
Abstract:
Language model pretraining has led to significant performance gains but careful comparison between different approaches is challenging. Training is computationally expensive, often done on private datasets of different sizes, and, as we will show, hyperparameter choices have significant impact on the final results. We present a replication study of BERT pretraining (Devlin et al., 2019) that carefully measures the impact of many key hyperparameters and training data size. We find that BERT was significantly undertrained, and can match or exceed the performance of every model published after it. Our best model achieves state-of-the-art results on GLUE, RACE and SQuAD. These results highlight the importance of previously overlooked design choices, and raise questions about the source of recently reported improvements. We release our models and code.
Authors: Yinhan Liu, Myle Ott, Naman Goyal, Jingfei Du, Mandar Joshi, Danqi Chen, Omer Levy, Mike Lewis, Luke Zettlemoyer, Veselin Stoyanov
https://arxiv.org/abs/1907.11692
YouTube: https://www.youtube.com/c/yannickilcher
Twitter: https://twitter.com/ykilcher
Minds: https://www.minds.com/ykilcher
BitChute: https://www.bitchute.com/channel/10a5ui845DOJ/
Видео RoBERTa: A Robustly Optimized BERT Pretraining Approach канала Yannic Kilcher
Показать
Комментарии отсутствуют
Информация о видео
Другие видео канала
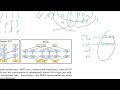
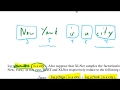
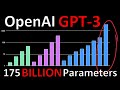
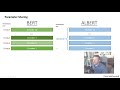
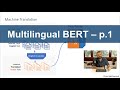
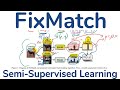
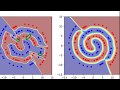

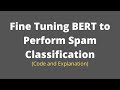
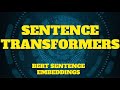
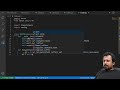
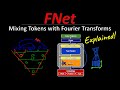
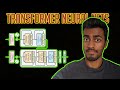
![[Rant] Can AI read your emotions? (No, but ...)](https://i.ytimg.com/vi/zWFkUGXjbdo/default.jpg)
![[Classic] Deep Residual Learning for Image Recognition (Paper Explained)](https://i.ytimg.com/vi/GWt6Fu05voI/default.jpg)
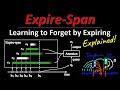
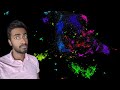
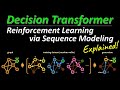
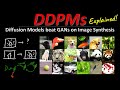
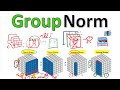