Jure Leskovec: "Large-scale Graph Representation Learning"
New Deep Learning Techniques 2018
"Large-scale Graph Representation Learning"
Jure Leskovec, Stanford University
Abstract: Machine learning on graphs is an important and ubiquitous task with applications ranging from drug design to friendship recommendation in social networks. The primary challenge in this domain is finding a way to represent, or encode, graph structure so that it can be easily exploited by machine learning models. However, traditionally machine learning approaches relied on user-defined heuristics to extract features encoding structural information about a graph. In this talk I will discuss methods that automatically learn to encode graph structure into low-dimensional embeddings, using techniques based on deep learning and nonlinear dimensionality reduction. I will provide a conceptual review of key advancements in this area of representation learning on graphs, including random-walk based algorithms, and graph convolutional networks.
Institute for Pure and Applied Mathematics, UCLA
February 7, 2018
For more information: http://www.ipam.ucla.edu/programs/workshops/new-deep-learning-techniques/?tab=overview
Видео Jure Leskovec: "Large-scale Graph Representation Learning" канала Institute for Pure & Applied Mathematics (IPAM)
"Large-scale Graph Representation Learning"
Jure Leskovec, Stanford University
Abstract: Machine learning on graphs is an important and ubiquitous task with applications ranging from drug design to friendship recommendation in social networks. The primary challenge in this domain is finding a way to represent, or encode, graph structure so that it can be easily exploited by machine learning models. However, traditionally machine learning approaches relied on user-defined heuristics to extract features encoding structural information about a graph. In this talk I will discuss methods that automatically learn to encode graph structure into low-dimensional embeddings, using techniques based on deep learning and nonlinear dimensionality reduction. I will provide a conceptual review of key advancements in this area of representation learning on graphs, including random-walk based algorithms, and graph convolutional networks.
Institute for Pure and Applied Mathematics, UCLA
February 7, 2018
For more information: http://www.ipam.ucla.edu/programs/workshops/new-deep-learning-techniques/?tab=overview
Видео Jure Leskovec: "Large-scale Graph Representation Learning" канала Institute for Pure & Applied Mathematics (IPAM)
Показать
Комментарии отсутствуют
Информация о видео
17 февраля 2018 г. 3:52:45
00:49:48
Другие видео канала
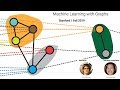
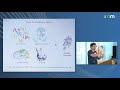
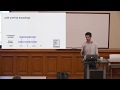
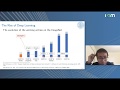
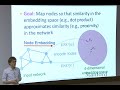
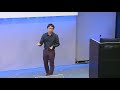
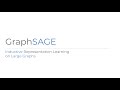
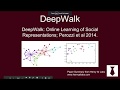
![[Original ResNet paper] Deep Residual Learning for Image Recognition | AISC](https://i.ytimg.com/vi/jio04YvgraU/default.jpg)
![[IPAM2019] Thomas Kipf "Unsupervised Learning with Graph Neural Networks"](https://i.ytimg.com/vi/9jSFBcptZ9A/default.jpg)
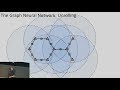
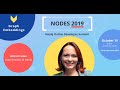
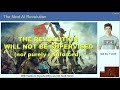
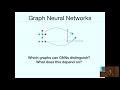
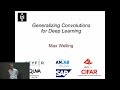
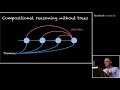
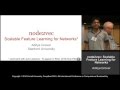
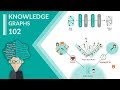

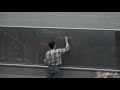