Deep Learning in Optics
Presented By:
Aydogan Ozcan, PhD - Professor, UCLA
Speaker Biography:
Dr. Ozcan is the Chancellor's Professor at UCLA and an HHMI Professor with the Howard Hughes Medical Institute, leading the Bio- and Nano-Photonics Laboratory at UCLA School of Engineering and is also the Associate Director of the California NanoSystems Institute.
Co-Presented By:
Hongda Wang, MS, BS - Graduate Student, UCLA
Speaker Biography:
Hongda Wang is a M.S./Ph.D. student of the Electrical and Computer Engineering Department at the University of California, Los Angeles (UCLA). He received his Bachelor of Science in Physics from the School of Physics in Peking University in 2015, and Master of Science in Electrical and Computer Engineering from the Henry Samueli School of Engineering and Applied Science in UCLA.
Co-Presented By:
Yair Rivenson, PhD - Adjunct Professor, UCLA
Speaker Biography:
Dr. Rivenson is an adjunct professor in the Department of Electrical and Computer Engineering, UCLA. His current research interests include computational imaging and sensing for biomedical, life sciences and environmental applications, and physics inspired machine learning.
Co-Presented By:
Laurent Bentolila, PhD - Research Scientist, UCLA
Speaker Biography:
Dr. Bentolila is Director of the Advanced Light Microscopy/Spectroscopy Laboratory, the Macro-Scale Imaging Laboratory, the Leica Microsystems Center of Excellence and a Research Scientist at the California NanoSystems Institute at UCLA. Dr. Bentolila's long-standing research interest focuses on the application of nanotechnology and advanced light microscopy techniques to biology and medicine.
Webinar:
Deep Learning in Optics
Webinar Abstract:
Researchers at the California NanoSystems Institute (CNSI) at UCLA have created a novel, data-driven, deep learning framework that allows for the generation of super-resolution images directly from images acquired on conventional, diffraction-limited microscopes. This is completed without prior knowledge about the sample and/or the image formation process to super-resolve microscopic images beyond the diffraction limit. The deep network output is extremely fast, without any iterations or parameter searches. In another demonstration, the researchers have used a deep neural network to perform virtual histological staining of a label free tissue sections, using a single autofluorescence image. This transformation, which uses only the endogenous contrast of the tissue section, was applied to multiple types of tissues and stains and blindly validated by board a panel of pathologists.
These results represent an important step towards computational microscopy and illustrate some of the potential machine learning to the field.
Learning Objectives:
-Understanding of computational microscopy principles
-Using deep learning algorithms to generate super-resolution images
-Using deep learning algorithms for virtual staining
Earn PACE Credits:
1. Make sure you’re a registered member of LabRoots (https://www.labroots.com/ms/virtual-event/molecular-diagnostics-2019)
2. Watch the Event’s webinar on YouTube or on the LabRoots Website (https://www.labroots.com/ms/virtual-event/molecular-diagnostics-2019)
3. Click Here to get your PACE credits (Expiration date – April 02, 2021 12:00 AM): https://www.labroots.com/credit/pace-credits/3436/third-party
LabRoots on Social:
Facebook: https://www.facebook.com/LabRootsInc
Twitter: https://twitter.com/LabRoots
LinkedIn: https://www.linkedin.com/company/labroots
Instagram: https://www.instagram.com/labrootsinc
Pinterest: https://www.pinterest.com/labroots/
SnapChat: labroots_inc
Видео Deep Learning in Optics канала Labroots
Aydogan Ozcan, PhD - Professor, UCLA
Speaker Biography:
Dr. Ozcan is the Chancellor's Professor at UCLA and an HHMI Professor with the Howard Hughes Medical Institute, leading the Bio- and Nano-Photonics Laboratory at UCLA School of Engineering and is also the Associate Director of the California NanoSystems Institute.
Co-Presented By:
Hongda Wang, MS, BS - Graduate Student, UCLA
Speaker Biography:
Hongda Wang is a M.S./Ph.D. student of the Electrical and Computer Engineering Department at the University of California, Los Angeles (UCLA). He received his Bachelor of Science in Physics from the School of Physics in Peking University in 2015, and Master of Science in Electrical and Computer Engineering from the Henry Samueli School of Engineering and Applied Science in UCLA.
Co-Presented By:
Yair Rivenson, PhD - Adjunct Professor, UCLA
Speaker Biography:
Dr. Rivenson is an adjunct professor in the Department of Electrical and Computer Engineering, UCLA. His current research interests include computational imaging and sensing for biomedical, life sciences and environmental applications, and physics inspired machine learning.
Co-Presented By:
Laurent Bentolila, PhD - Research Scientist, UCLA
Speaker Biography:
Dr. Bentolila is Director of the Advanced Light Microscopy/Spectroscopy Laboratory, the Macro-Scale Imaging Laboratory, the Leica Microsystems Center of Excellence and a Research Scientist at the California NanoSystems Institute at UCLA. Dr. Bentolila's long-standing research interest focuses on the application of nanotechnology and advanced light microscopy techniques to biology and medicine.
Webinar:
Deep Learning in Optics
Webinar Abstract:
Researchers at the California NanoSystems Institute (CNSI) at UCLA have created a novel, data-driven, deep learning framework that allows for the generation of super-resolution images directly from images acquired on conventional, diffraction-limited microscopes. This is completed without prior knowledge about the sample and/or the image formation process to super-resolve microscopic images beyond the diffraction limit. The deep network output is extremely fast, without any iterations or parameter searches. In another demonstration, the researchers have used a deep neural network to perform virtual histological staining of a label free tissue sections, using a single autofluorescence image. This transformation, which uses only the endogenous contrast of the tissue section, was applied to multiple types of tissues and stains and blindly validated by board a panel of pathologists.
These results represent an important step towards computational microscopy and illustrate some of the potential machine learning to the field.
Learning Objectives:
-Understanding of computational microscopy principles
-Using deep learning algorithms to generate super-resolution images
-Using deep learning algorithms for virtual staining
Earn PACE Credits:
1. Make sure you’re a registered member of LabRoots (https://www.labroots.com/ms/virtual-event/molecular-diagnostics-2019)
2. Watch the Event’s webinar on YouTube or on the LabRoots Website (https://www.labroots.com/ms/virtual-event/molecular-diagnostics-2019)
3. Click Here to get your PACE credits (Expiration date – April 02, 2021 12:00 AM): https://www.labroots.com/credit/pace-credits/3436/third-party
LabRoots on Social:
Facebook: https://www.facebook.com/LabRootsInc
Twitter: https://twitter.com/LabRoots
LinkedIn: https://www.linkedin.com/company/labroots
Instagram: https://www.instagram.com/labrootsinc
Pinterest: https://www.pinterest.com/labroots/
SnapChat: labroots_inc
Видео Deep Learning in Optics канала Labroots
Показать
Комментарии отсутствуют
Информация о видео
Другие видео канала
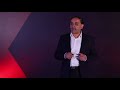

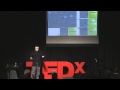
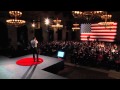
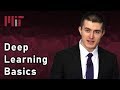
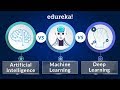

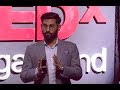
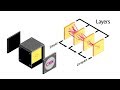

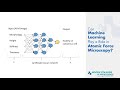

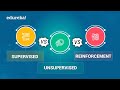
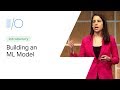
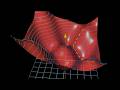
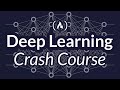
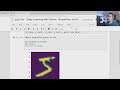
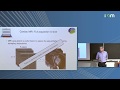
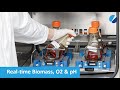
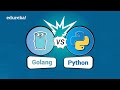